
Our Research
The Department of “Computational Biology for Infection Research” studies the human microbiome, viral and bacterial pathogens, and human cell lineages within individual patients by analysis of large-scale biological and epidemiological data sets with computational techniques. Focusing on high throughput meta’omics, population genomic and single cell sequencing data, we produce testable hypotheses, such as sets of key sites or relevant genes implicated in onset of a disease, antibiotic resistance or immune defense. We interact with experimental collaborators to verify our findings and to promote their translation into medical treatment or diagnosis procedures. To achieve its research goals, the department also develops novel algorithms and software.
Our Research
The Department of “Computational Biology for Infection Research” studies the human microbiome, viral and bacterial pathogens, and human cell lineages within individual patients by analysis of large-scale biological and epidemiological data sets with computational techniques. Focusing on high throughput meta’omics, population genomic and single cell sequencing data, we produce testable hypotheses, such as sets of key sites or relevant genes implicated in onset of a disease, antibiotic resistance or immune defense. We interact with experimental collaborators to verify our findings and to promote their translation into medical treatment or diagnosis procedures. To achieve its research goals, the department also develops novel algorithms and software.
Prof Dr Alice McHardy
Alice Carolyn McHardy holds a diploma in biochemistry and a doctoral degree (Dr. rer. nat) in bioinformatics, both from Bielefeld University in Germany. From 2005 to 2007 she first was a postdoc and then a permanent staff member in the Bioinformatics and Pattern Discovery Group at the IBM T.J. Watson Research Center in Yorktown Heights, USA.
She then became the head of the independent research group for Computational Genomics and Epidemiology at the Max Planck Institute of Computer Science in Saarbrücken. In 2010, she was appointed Chair of Algorithmic Bioinformatics at Heinrich Heine University in Düsseldorf.
In 2014, she became head of the Department of Computational Biology for Infection Research at the Helmholtz Centre for Infection Research in Braunschweig and was appointed as a full professor at TU Braunschweig.
Team

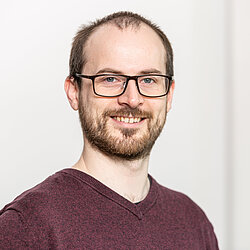
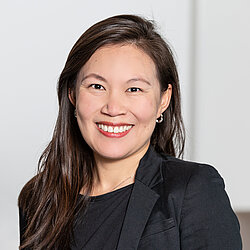
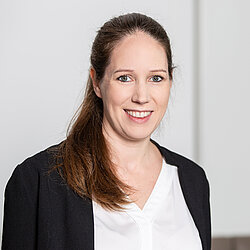
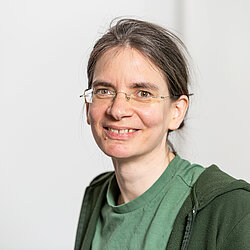

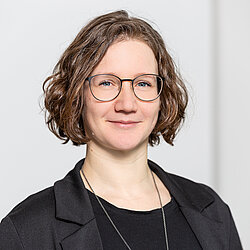

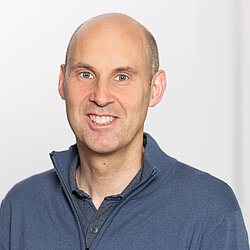
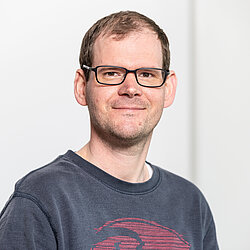
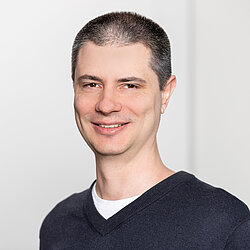
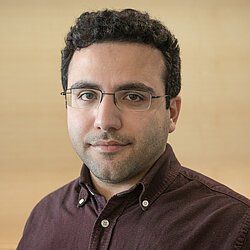
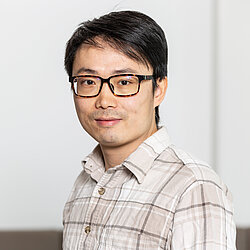
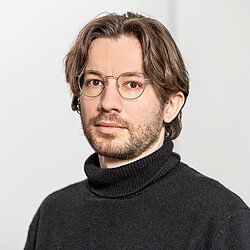
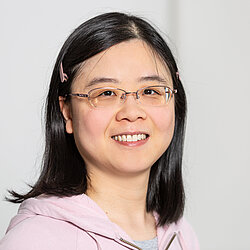
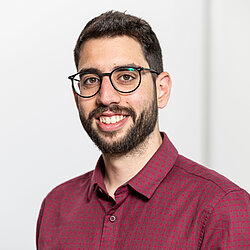
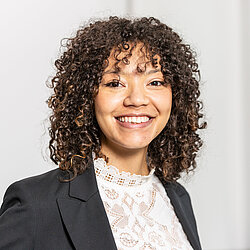

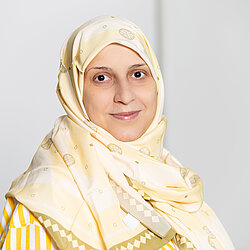
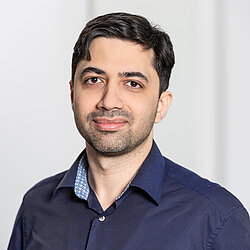

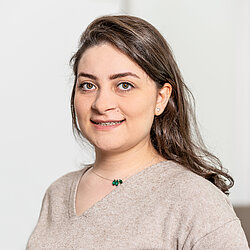
Web Applications
Traitar is a web service for phenotyping bacteria based on their genome sequences.
PhyloPythiaS+ - a self-training method for the rapid reconstruction of low-ranking taxonomic bins from metagenomes
PhyloPythiaS - the PhyloPythiaS Web Server for Taxonomic Assignment of Metagenome Sequences.
Taxator-tk performs taxonomic sequence assignment by fast approximate determination of evolutionary neighbors from sequence similarities.
AdaPatch is a method for detecting positively selected patches of sites on the surface of viral proteins, which are likely candidates for being relevant for adaptive evolution.
Software Downloads
Software implementing the group’s research can be downloaded here or on GitHub at github.com/hzi-bifo, such as
SDplots: software for the detection of selective sweeps to monitor the adaptation of influenza A viruses
SDplots VaccineUpdates: results of the bi-annual vaccine strain prediction for influenza A viruses
PatchDetection: software for the detection of protein patches under positive selection
Phylogeography: software for phylogeographical reconstruction to infer origin and spread routes of viral pathogen outbreaks
FrechetTreeDistances: distances between phylogeographic reconstructions across tree topologies.
DiTaxa: nucleotide-pair encoding of 16S rRNA sequences for host phenotype and biomarker detection
CAMISIM: Simulating metagenomes and microbial communities
AMBER: Assessment of Metagenome BinnERs
OPAL: Open-community Profiling Assessment tooL
CAMITAX: Taxon labels for microbial genomes
Selected Publications
- Meyer, F., Fritz, A., Deng, Z. L., Koslicki, D., Lesker, T. R., Gurevich, A., Robertson, G., Alser, M., Antipov, D., Beghini, F., Bertrand, D., Brito, J. J., Brown, C. T., Buchmann, J., Buluç, A., Chen, B., Chikhi, R., Clausen, P., Cristian, A., Dabrowski, P. W., Darling, A. E., Egan, R., Eskin, E., Georganas, E., Goltsman, E., Gray, M. A., Hansen, L. H., Hofmeyr, S., Huang, P., Irber, L., Jia, H., Jørgensen, T. S., Kieser, S. D., Klemetsen, T., Kola, A., Kolmogorov, M., Korobeynikov, A., Kwan, J., LaPierre, N., Lemaitre, C., Li, C., Limasset, A., Malcher-Miranda, F., Mangul, S., Marcelino, V. R., Marchet, C., Marijon, P., Meleshko, D., Mende, D. R., Milanese, A., Nagarajan, N., Nissen, J., Nurk, S., Oliker, L., Paoli, L., Peterlongo, P., Piro, V. C., Porter, J. S., Rasmussen, S., Rees, E. R., Reinert, K., Renard, B., Robertsen, E. M., Rosen, G. L., Ruscheweyh, H. J., Sarwal, V., Segata, N., Seiler, E., Shi, L., Sun, F., Sunagawa, S., Sørensen, S. J., Thomas, A., Tong, C., Trajkovski, M., Tremblay, J., Uritskiy, G., Vicedomini, R., Wang, Z., Wang, Z., Wang, Z., Warren, A., Willassen, N. P., Yelick, K., You, R., Zeller, G., Zhao, Z., Zhu, S., Zhu, J., Garrido-Oter, R., Gastmeier, P., Hacquard, S., Häußler, S., Khaledi, A., Maechler, F., Mesny, F., Radutoiu, S., Schulze-Lefert, P., Smit, N., Strowig, T., Bremges, A., Sczyrba, A. & McHardy, A. C. Critical Assessment of Metagenome Interpretation: the second round of challenges. Nat Methods (2022) 19, 429, doi:10.1038/s41592-022-01431-4.
- Asgari, E., Münch, P. C., Lesker, T. R., McHardy, A. C.* & Mofrad, M. R. K.* (*shared last authors) DiTaxa: nucleotide-pair encoding of 16S rRNA for host phenotype and biomarker detection. Bioinformatics (2019) 35, 2498, doi:10.1093/bioinformatics/bty954.
- Bankwitz, D.*, Bahai, A.*, Labuhn, M., Doepke, M., Ginkel, C., Khera, T., Todt, D., Ströh, L. J., Dold, L., Klein, F., Klawonn, F., Krey, T., Behrendt, P., Cornberg, M., McHardy, A. C.* & Pietschmann, T*. (*shared first and last authors) Hepatitis C reference viruses highlight potent antibody responses and diverse viral functional interactions with neutralising antibodies. Gut (2021) 70, 1734, doi:10.1136/gutjnl-2020-321190.
- Fritz, A.*, Hofmann, P.*, Majda, S., Dahms, E., Dröge, J., Fiedler, J., Lesker, T. R., Belmann, P., DeMaere, M. Z., Darling, A. E., Sczyrba, A., Bremges, A. & McHardy, A. C. (*shared first authors) CAMISIM: simulating metagenomes and microbial communities. Microbiome (2019) 7, 17, doi:10.1186/s40168-019-0633-6.
- Münch, P. C., Franzosa, E. A., Stecher, B., McHardy, A. C.* & Huttenhower, C.* (*shared last authors) Identification of Natural CRISPR Systems and Targets in the Human Microbiome. Cell Host Microbe (2021) 29, 94, doi:10.1016/j.chom.2020.10.010.
Publications
2022
- Poussin, C., Khachatryan, L., Sierro, N., Narsapuram, V.K., Meyer, F., Kaikala, V., Chawla, V., Muppirala, U., Kumar, S., Belcastro, V., Battey, JND., Scotti, E., Boué, S., McHardy, A.C., Peitsch, M.C., Ivanov, N.V., Hoeng, J. Crowdsourced benchmarking of taxonomic metagenome profilers: lessons learned from the sbv IMPROVER Microbiomics challenge. BMC Genomics (2022), 23, 624, doi: 10.1186/s12864-022-08803-2.
- Cernava, T., Rybakova, D., Buscot, F., Clavel, T., McHardy, A.C., Meyer, F., Overmann, J., Stecher, B., Sessitsch, A., Schloter, M., Berg, G. Metadata harmonization-Standards are the key for a better usage of omics data for integrative microbiome analysis. Environ Microbiome (2022), 17, 33, doi: 10.1186/s40793-022-00425-1.
- Foroughmand-Araabi, M.H., Goliaei, S., McHardy, A.C. Scelestial: Fast and accurate single-cell lineage tree inference based on Steiner tree approximation algorithm. PLoS Comput Biol (2022), 18, e1009100, doi: 10.1371/journal.pcbi.1009100.
- Ghazi, A. R., Münch, P. C., Chen, D., Jensen, J. & Huttenhower, C. Strain Identification and Quantitative Analysis in Microbial Communities. J Mol Biol (2022) 434, 167582, doi:10.1016/j.jmb.2022.167582.
- Deng, Z. L., Münch, P. C., Mreches, R. & McHardy, A. C. Rapid and accurate identification of ribosomal RNA sequences via deep learning. Nucleic Acids Res (2022) 50, e60, doi:10.1093/nar/gkac112.
- Hezaveh, K., Shinde, R. S., Klötgen, A., Halaby, M. J., Lamorte, S., Ciudad, M. T., Quevedo, R., Neufeld, L., Liu, Z. Q., Jin, R., Grünwald, B. T., Foerster, E. G., Chaharlangi, D., Guo, M., Makhijani, P., Zhang, X., Pugh, T. J., Pinto, D. M., Co, I. L., McGuigan, A. P., Jang, G. H., Khokha, R., Ohashi, P. S., O'Kane, G. M., Gallinger, S., Navarre, W. W., Maughan, H., Philpott, D. J., Brooks, D. G. & McGaha, T. L. Tryptophan-derived microbial metabolites activate the aryl hydrocarbon receptor in tumor-associated macrophages to suppress anti-tumor immunity. Immunity (2022) 55, 324, doi:10.1016/j.immuni.2022.01.006.
- Papafragkos, I., Grigoriou, M., Boon, L., Kloetgen, A., Hatzioannou, A. & Verginis, P. Ablation of NLRP3 inflammasome rewires MDSC function and promotes tumor regression. Front Immunol (2022) 13, 889075, doi:10.3389/fimmu.2022.889075.
- Thandapani, P., Kloetgen, A., Witkowski, M. T., Glytsou, C., Lee, A. K., Wang, E., Wang, J., LeBoeuf, S. E., Avrampou, K., Papagiannakopoulos, T., Tsirigos, A. & Aifantis, I. Valine tRNA levels and availability regulate complex I assembly in leukaemia. Nature (2022) 601, 428, doi:10.1038/s41586-021-04244-1.
- Weiss, A. S., Burrichter, A. G., Durai Raj, A. C., von Strempel, A., Meng, C., Kleigrewe, K., Münch, P. C., Rössler, L., Huber, C., Eisenreich, W., Jochum, L. M., Göing, S., Jung, K., Lincetto, C., Hübner, J., Marinos, G., Zimmermann, J., Kaleta, C., Sanchez, A. & Stecher, B. In vitro interaction network of a synthetic gut bacterial community. Isme j (2022) 16, 1095, doi:10.1038/s41396-021-01153-z.
- Zickler, M., Stanelle-Bertram, S., Ehret, S., Heinrich, F., Lange, P., Schaumburg, B., Kouassi, N. M., Beck, S., Jaeckstein, M. Y., Mann, O., Krasemann, S., Schroeder, M., Jarczak, D., Nierhaus, A., Kluge, S., Peschka, M., Schlüter, H., Renné, T., Pueschel, K., Kloetgen, A., Scheja, L., Ondruschka, B., Heeren, J. & Gabriel, G. Replication of SARS-CoV-2 in adipose tissue determines organ and systemic lipid metabolism in hamsters and humans. Cell Metab (2022) 34, 1, doi:10.1016/j.cmet.2021.12.002.
- Houwaart, T., Belhaj, S., Tawalbeh, E., Nagels, D., Fröhlich, Y., Finzer, P., Ciruela, P., Sabrià, A., Herrero, M., Andrés, C., Antón, A., Benmoumene, A., Asskali, D., Haidar, H., von Dahlen, J., Nicolai, J., Stiller, M., Blum, J., Lange, C., Adelmann, C., Schroer, B., Osmers, U., Grice, C., Kirfel, P. P., Jomaa, H., Strelow, D., Hülse, L., Pigulla, M., Kreuzer, P., Tyshaieva, A., Weber, J., Wienemann, T., Kohns Vasconcelos, M., Hoffmann, K., Lübke, N., Hauka, S., Andree, M., Scholz, C. J., Jazmati, N., Göbels, K., Zotz, R., Pfeffer, K., Timm, J., Ehlkes, L., Walker, A. & Dilthey, A. T. Integrated genomic surveillance enables tracing of person-to-person SARS-CoV-2 transmission chains during community transmission and reveals extensive onward transmission of travel-imported infections, Germany, June to July 2021. Euro Surveill (2022) 27, doi:10.2807/1560-7917.Es.2022.27.43.2101089.
- Hotop, S. K., Reimering, S., Shekhar, A., Asgari, E., Beutling, U., Dahlke, C., Fathi, A., Khan, F., Lütgehetmann, M., Ballmann, R., Gerstner, A., Tegge, W., Cicin-Sain, L., Bilitewski, U., McHardy, A. C. & Brönstrup, M. Peptide microarrays coupled to machine learning reveal individual epitopes from human antibody responses with neutralizing capabilities against SARS-CoV-2. Emerg Microbes Infect (2022), 11, 1037, doi:10.1080/22221751.2022.2057874.
- McHardy, A. C. Nobel nominators - which women will you suggest? Nature (2022) 601, 508, doi:10.1038/d41586-022-00133-3.
- Meyer, F.*, Fritz, A.*, Deng, Z. L., Koslicki, D., Lesker, T. R., Gurevich, A., Robertson, G., Alser, M., Antipov, D., Beghini, F., Bertrand, D., Brito, J. J., Brown, C. T., Buchmann, J., Buluç, A., Chen, B., Chikhi, R., Clausen, P., Cristian, A., Dabrowski, P. W., Darling, A. E., Egan, R., Eskin, E., Georganas, E., Goltsman, E., Gray, M. A., Hansen, L. H., Hofmeyr, S., Huang, P., Irber, L., Jia, H., Jørgensen, T. S., Kieser, S. D., Klemetsen, T., Kola, A., Kolmogorov, M., Korobeynikov, A., Kwan, J., LaPierre, N., Lemaitre, C., Li, C., Limasset, A., Malcher-Miranda, F., Mangul, S., Marcelino, V. R., Marchet, C., Marijon, P., Meleshko, D., Mende, D. R., Milanese, A., Nagarajan, N., Nissen, J., Nurk, S., Oliker, L., Paoli, L., Peterlongo, P., Piro, V. C., Porter, J. S., Rasmussen, S., Rees, E. R., Reinert, K., Renard, B., Robertsen, E. M., Rosen, G. L., Ruscheweyh, H. J., Sarwal, V., Segata, N., Seiler, E., Shi, L., Sun, F., Sunagawa, S., Sørensen, S. J., Thomas, A., Tong, C., Trajkovski, M., Tremblay, J., Uritskiy, G., Vicedomini, R., Wang, Z., Wang, Z., Wang, Z., Warren, A., Willassen, N. P., Yelick, K., You, R., Zeller, G., Zhao, Z., Zhu, S., Zhu, J., Garrido-Oter, R., Gastmeier, P., Hacquard, S., Häußler, S., Khaledi, A., Maechler, F., Mesny, F., Radutoiu, S., Schulze-Lefert, P., Smit, N., Strowig, T., Bremges, A., Sczyrba, A. & McHardy, A. C. (*shared first authors) Critical Assessment of Metagenome Interpretation: the second round of challenges. Nat Methods (2022) 19, 429, doi:10.1038/s41592-022-01431-4.
2021
- Goeser, F., Münch, P., Lesker, T. R., Lutz, P. L., Krämer, B., Kaczmarek, D. J., Finnemann, C., Nischalke, H. D., Geffers, R., Parcina, M., McHardy, A. C., Strassburg, C., Hoerauf, A., Nattermann, J., Bekeredjian-Ding, I., & Spengler, U. Neither black nor white: do altered intestinal microbiota reflect chronic liver disease severity? Gut(2021) 438, 440. doi.org/10.1136/gutjnl-2020-321424
- Yusufova, N., Kloetgen, A., Teater, M., Osunsade, A., Camarillo, J. M., Chin, C. R., Doane, A. S., Venters, B. J., Portillo-Ledesma, S., Conway, J., Phillip, J. M., Elemento, O., Scott, D. W., Béguelin, W., Licht, J. D., Kelleher, N. L., Staudt, L. M., Skoultchi, A. I., Keogh, M. C., Apostolou, E., Mason, C. E., Imielinski, M., Schlick, T., David, Y., Tsirigos, A., Allis, C. D., Soshnev, A. A., Cesarman, E. & Melnick, A. M. Histone H1 loss drives lymphoma by disrupting 3D chromatin architecture. Nature (2021) 589, 299, doi:10.1038/s41586-020-3017-y.
- Weiss, A. S., Burrichter, A. G., Durai Raj, A. C., von Strempel, A., Meng, C., Kleigrewe, K., Münch, P. C., Rössler, L., Huber, C., Eisenreich, W., Jochum, L. M., Göing, S., Jung, K., Lincetto, C., Hübner, J., Marinos, G., Zimmermann, J., Kaleta, C., Sanchez, A. & Stecher, B. In vitro interaction network of a synthetic gut bacterial community. Isme j (2021) in press, doi:10.1038/s41396-021-01153-z.
- Wang, E., Zhou, H., Nadorp, B., Cayanan, G., Chen, X., Yeaton, A. H., Nomikou, S., Witkowski, M. T., Narang, S., Kloetgen, A., Thandapani, P., Ravn-Boess, N., Tsirigos, A. & Aifantis, I. Surface antigen-guided CRISPR screens identify regulators of myeloid leukemia differentiation. Cell Stem Cell (2021) 28, 718, doi:10.1016/j.stem.2020.12.005.
- Warnat-Herresthal, S., Schultze, H., Shastry, K. L., Manamohan, S., Mukherjee, S., Garg, V., Sarveswara, R., Händler, K., Pickkers, P., Aziz, N. A., Ktena, S., Tran, F., Bitzer, M., Ossowski, S., Casadei, N., Herr, C., Petersheim, D., Behrends, U., Kern, F., Fehlmann, T., Schommers, P., Lehmann, C., Augustin, M., Rybniker, J., Altmüller, J., Mishra, N., Bernardes, J. P., Krämer, B., Bonaguro, L., Schulte-Schrepping, J., De Domenico, E., Siever, C., Kraut, M., Desai, M., Monnet, B., Saridaki, M., Siegel, C. M., Drews, A., Nuesch-Germano, M., Theis, H., Heyckendorf, J., Schreiber, S., Kim-Hellmuth, S., Nattermann, J., Skowasch, D., Kurth, I., Keller, A., Bals, R., Nürnberg, P., Rieß, O., Rosenstiel, P., Netea, M. G., Theis, F., Mukherjee, S., Backes, M., Aschenbrenner, A. C., Ulas, T., Breteler, M. M. B., Giamarellos-Bourboulis, E. J., Kox, M., Becker, M., Cheran, S., Woodacre, M. S., Goh, E. L. & Schultze, J. L. Swarm Learning for decentralized and confidential clinical machine learning. Nature (2021) 594, 265, doi:10.1038/s41586-021-03583-3.
- Rivas, M. A., Meydan, C., Chin, C. R., Challman, M. F., Kim, D., Bhinder, B., Kloetgen, A., Viny, A. D., Teater, M. R., McNally, D. R., Doane, A. S., Béguelin, W., Fernández, M. T. C., Shen, H., Wang, X., Levine, R. L., Chen, Z., Tsirigos, A., Elemento, O., Mason, C. E. & Melnick, A. M. Smc3 dosage regulates B cell transit through germinal centers and restricts their malignant transformation. Nat Immunol (2021) 22, 240, doi:10.1038/s41590-020-00827-8.
- Rivas, M. A., Durmaz, C., Kloetgen, A., Chin, C. R., Chen, Z., Bhinder, B., Koren, A., Viny, A. D., Scharer, C. D., Boss, J. M., Elemento, O., Mason, C. E. & Melnick, A. M. Cohesin Core Complex Gene Dosage Contributes to Germinal Center Derived Lymphoma Phenotypes and Outcomes. Front Immunol (2021) 12, 688493, doi:10.3389/fimmu.2021.688493.
- Pelham-Webb, B., Polyzos, A., Wojenski, L., Kloetgen, A., Li, J., Di Giammartino, D. C., Sakellaropoulos, T., Tsirigos, A., Core, L. & Apostolou, E. H3K27ac bookmarking promotes rapid post-mitotic activation of the pluripotent stem cell program without impacting 3D chromatin reorganization. Mol Cell (2021) 81, 1732, doi:10.1016/j.molcel.2021.02.032.
- Münch, P. C., Franzosa, E. A., Stecher, B., McHardy, A. C.* & Huttenhower, C.* (*shared last authors) Identification of Natural CRISPR Systems and Targets in the Human Microbiome. Cell Host Microbe (2021) 29, 94, doi:10.1016/j.chom.2020.10.010.
- Mirzayi, C., Renson, A., Zohra, F., Elsafoury, S., Geistlinger, L., Kasselman, L. J., Eckenrode, K., van de Wijgert, J., Loughman, A., Marques, F. Z., MacIntyre, D. A., Arumugam, M., Azhar, R., Beghini, F., Bergstrom, K., Bhatt, A., Bisanz, J. E., Braun, J., Bravo, H. C., Buck, G. A., Bushman, F., Casero, D., Clarke, G., Collado, M. C., Cotter, P. D., Cryan, J. F., Demmer, R. T., Devkota, S., Elinav, E., Escobar, J. S., Fettweis, J., Finn, R. D., Fodor, A. A., Forslund, S., Franke, A., Furlanello, C., Gilbert, J., Grice, E., Haibe-Kains, B., Handley, S., Herd, P., Holmes, S., Jacobs, J. P., Karstens, L., Knight, R., Knights, D., Koren, O., Kwon, D. S., Langille, M., Lindsay, B., McGovern, D., McHardy, A. C., McWeeney, S., Mueller, N. T., Nezi, L., Olm, M., Palm, N., Pasolli, E., Raes, J., Redinbo, M. R., Rühlemann, M., Balfour Sartor, R., Schloss, P. D., Schriml, L., Segal, E., Shardell, M., Sharpton, T., Smirnova, E., Sokol, H., Sonnenburg, J. L., Srinivasan, S., Thingholm, L. B., Turnbaugh, P. J., Upadhyay, V., Walls, R. L., Wilmes, P., Yamada, T., Zeller, G., Zhang, M., Zhao, N., Zhao, L., Bao, W., Culhane, A., Devanarayan, V., Dopazo, J., Fan, X., Fischer, M., Jones, W., Kusko, R., Mason, C. E., Mercer, T. R., Sansone, S. A., Scherer, A., Shi, L., Thakkar, S., Tong, W., Wolfinger, R., Hunter, C., Segata, N., Huttenhower, C., Dowd, J. B., Jones, H. E. & Waldron, L. Reporting guidelines for human microbiome research: the STORMS checklist. Nat Med (2021) 27, 1885, doi:10.1038/s41591-021-01552-x.
- Meyer, F., Lesker, T. R., Koslicki, D., Fritz, A., Gurevich, A., Darling, A. E., Sczyrba, A., Bremges, A. & McHardy, A. C. Tutorial: assessing metagenomics software with the CAMI benchmarking toolkit. Nat Protoc (2021) 16, 1785, doi:10.1038/s41596-020-00480-3.
- Lähnemann, D., Köster, J., Fischer, U., Borkhardt, A., McHardy, A. C.* & Schönhuth, A.* (*shared last authors) Accurate and scalable variant calling from single cell DNA sequencing data with ProSolo. Nat Commun (2021) 12, 6744, doi:10.1038/s41467-021-26938-w.
- Krämer, B., Knoll, R., Bonaguro, L., ToVinh, M., Raabe, J., Astaburuaga-García, R., Schulte-Schrepping, J., Kaiser, K. M., Rieke, G. J., Bischoff, J., Monin, M. B., Hoffmeister, C., Schlabe, S., De Domenico, E., Reusch, N., Händler, K., Reynolds, G., Blüthgen, N., Hack, G., Finnemann, C., Nischalke, H. D., Strassburg, C. P., Stephenson, E., Su, Y., Gardner, L., Yuan, D., Chen, D., Goldman, J., Rosenstiel, P., Schmidt, S. V., Latz, E., Hrusovsky, K., Ball, A. J., Johnson, J. M., Koenig, P. A., Schmidt, F. I., Haniffa, M., Heath, J. R., Kümmerer, B. M., Keitel, V., Jensen, B., Stubbemann, P., Kurth, F., Sander, L. E., Sawitzki, B., Aschenbrenner, A. C., Schultze, J. L. & Nattermann, J. Early IFN-α signatures and persistent dysfunction are distinguishing features of NK cells in severe COVID-19. Immunity (2021) 54, 2650, doi:10.1016/j.immuni.2021.09.002.
- Jacobsen, H., Walendy-Gnirß, K., Tekin-Bubenheim, N., Kouassi, N. M., Ben-Batalla, I., Berenbrok, N., Wolff, M., Dos Reis, V. P., Zickler, M., Scholl, L., Gries, A., Jania, H., Kloetgen, A., Düsedau, A., Pilnitz-Stolze, G., Jeridi, A., Yildirim, A., Fuchs, H., Gailus-Durner, V., Stoeger, C., de Angelis, M. H., Manuylova, T., Klingel, K., Culley, F. J., Behrends, J., Loges, S., Schneider, B., Krauss-Etschmann, S., Openshaw, P. & Gabriel, G. Offspring born to influenza A virus infected pregnant mice have increased susceptibility to viral and bacterial infections in early life. Nat Commun (2021) 12, 4957, doi:10.1038/s41467-021-25220-3.
- Hufsky, F., Lamkiewicz, K., Almeida, A., Aouacheria, A., Arighi, C., Bateman, A., Baumbach, J., Beerenwinkel, N., Brandt, C., Cacciabue, M., Chuguransky, S., Drechsel, O., Finn, R. D., Fritz, A., Fuchs, S., Hattab, G., Hauschild, A. C., Heider, D., Hoffmann, M., Hölzer, M., Hoops, S., Kaderali, L., Kalvari, I., von Kleist, M., Kmiecinski, R., Kühnert, D., Lasso, G., Libin, P., List, M., Löchel, H. F., Martin, M. J., Martin, R., Matschinske, J., McHardy, A. C., Mendes, P., Mistry, J., Navratil, V., Nawrocki, E. P., O'Toole Á, N., Ontiveros-Palacios, N., Petrov, A. I., Rangel-Pineros, G., Redaschi, N., Reimering, S., Reinert, K., Reyes, A., Richardson, L., Robertson, D. L., Sadegh, S., Singer, J. B., Theys, K., Upton, C., Welzel, M., Williams, L. & Marz, M. Computational strategies to combat COVID-19: useful tools to accelerate SARS-CoV-2 and coronavirus research. Brief Bioinform (2021) 22, 642, doi:10.1093/bib/bbaa232.
- Grigoriou, M., Banos, A., Hatzioannou, A., Kloetgen, A., Kouzis, P., Aggouraki, D., Zakopoulou, R., Bamias, G., Kassi, E., Mavroudis, D., Bamias, A., Boumpas, D. T., Tsirigos, A., Gogas, H., Alissafi, T. & Verginis, P. Regulatory T-cell Transcriptomic Reprogramming Characterizes Adverse Events by Checkpoint Inhibitors in Solid Tumors. Cancer Immunol Res (2021) 9, 726, doi:10.1158/2326-6066.Cir-20-0969.
- Fritz, A., Bremges, A., Deng, Z. L., Lesker, T. R., Götting, J., Ganzenmueller, T., Sczyrba, A., Dilthey, A., Klawonn, F. & McHardy, A. C.Haploflow: strain-resolved de novo assembly of viral genomes. Genome Biol (2021) 22, 212, doi:10.1186/s13059-021-02426-8.
- Deng, Z. L., Dhingra, A., Fritz, A., Götting, J., Münch, P. C., Steinbrück, L., Schulz, T. F., Ganzenmüller, T. & McHardy, A. C. Evaluating assembly and variant calling software for strain-resolved analysis of large DNA viruses. Brief Bioinform (2021) 22, doi:10.1093/bib/bbaa123.
- Bankwitz, D.*, Bahai, A.*, Labuhn, M., Doepke, M., Ginkel, C., Khera, T., Todt, D., Ströh, L. J., Dold, L., Klein, F., Klawonn, F., Krey, T., Behrendt, P., Cornberg, M., McHardy, A. C.* & Pietschmann, T*. (*shared first and last authors) Hepatitis C reference viruses highlight potent antibody responses and diverse viral functional interactions with neutralising antibodies. Gut (2021) 70, 1734, doi:10.1136/gutjnl-2020-321190.
- Bahai, A., Asgari, E., Mofrad, M. R. K., Kloetgen, A. & McHardy, A. C. EpitopeVec: Linear Epitope Prediction Using Deep Protein Sequence Embeddings. Bioinformatics (2021) 37, 23, doi:10.1093/bioinformatics/btab467.
- Aronson, B. E., Scourzic, L., Shah, V., Swanzey, E., Kloetgen, A., Polyzos, A., Sinha, A., Azziz, A., Caspi, I., Li, J., Pelham-Webb, B., Glenn, R. A., Vierbuchen, T., Wichterle, H., Tsirigos, A., Dawlaty, M. M., Stadtfeld, M. & Apostolou, E. A bipartite element with allele-specific functions safeguards DNA methylation imprints at the Dlk1-Dio3 locus. Dev Cell (2021) 56, 3052, doi:10.1016/j.devcel.2021.10.004.
2020
- Bankwitz, D.*, Bahai, A.*, Labuhn, M., Doepke, M., Ginkel, C., Khera, T., Todt, D., Ströh, L. J., Dold, L., Klein, F., Klawonn, F., Krey, T., Behrendt, P., Cornberg, M., McHardy, A. C.* & Pietschmann, T.* (*shared first & last authors) Hepatitis C reference viruses highlight potent antibody responses and diverse viral functional interactions with neutralising antibodies. Gut (2020) in press, gutjnl-2020-321190, doi:10.1136/gutjnl-2020-321190.
- Bremges, A., Fritz, A. & McHardy, A. C. CAMITAX: Taxon labels for microbial genomes. Gigascience (2020) 9, giz154, doi:10.1093/gigascience/giz154.
- Deng, Z.-L., Dhingra, A., Fritz, A., Götting, J., Münch, P. C., Steinbrück, L., Schulz, T. F., Ganzenmüller, T. & McHardy, A. C. Evaluating assembly and variant calling software for strain-resolved analysis of large DNA viruses. Briefings in Bioinformatics (2020) in press, bbaa123, doi:10.1093/bib/bbaa123.
- Eberl C., Ring D., Münch P.C., Beutler M., Basic M., Slack E.C., Schwarzer M., Srutkova D., Lange A., Frick J.S., Bleich A., Stecher B. , Reproducible Colonization of Germ-Free Mice With the Oligo-Mouse-Microbiota in Different Animal Facilities. Frontiers in Microbiology (2020) 10, 2999, doi:10.3389/fmicb.2019.02999.
- Hanniford, D., Ulloa-Morales, A., Karz, A., Berzoti-Coelho, M. G., Moubarak, R. S., Sánchez-Sendra, B., Kloetgen, A., Davalos, V., Imig, J., Wu, P., Vasudevaraja, V., Argibay, D., Lilja, K., Tabaglio, T., Monteagudo, C., Guccione, E., Tsirigos, A., Osman, I., Aifantis, I. & Hernando, E. Epigenetic Silencing of CDR1as Drives IGF2BP3-Mediated Melanoma Invasion and Metastasis. Cancer Cell (2020) 37, 55, doi:10.1016/j.ccell.2019.12.007.
- Hufsky, F., Lamkiewicz, K., Almeida, A., Aouacheria, A., Arighi, C., Bateman, A., Baumbach, J., Beerenwinkel, N., Brandt, C., Cacciabue, M., Chuguransky, S., Drechsel, O., Finn, R. D., Fritz, A., Fuchs, S., Hattab, G., Hauschild, A. C., Heider, D., Hoffmann, M., Hölzer, M., Hoops, S., Kaderali, L., Kalvari, I., von Kleist, M., Kmiecinski, R., Kühnert, D., Lasso, G., Libin, P., List, M., Löchel, H. F., Martin, M. J., Martin, R., Matschinske, J., McHardy, A. C., Mendes, P., Mistry, J., Navratil, V., Nawrocki, E. P., O'Toole Á, N., Ontiveros-Palacios, N., Petrov, A. I., Rangel-Pineros, G., Redaschi, N., Reimering, S., Reinert, K., Reyes, A., Richardson, L., Robertson, D. L., Sadegh, S., Singer, J. B., Theys, K., Upton, C., Welzel, M., Williams, L. & Marz, M. Computational strategies to combat COVID-19: useful tools to accelerate SARS-CoV-2 and coronavirus research. Brief Bioinform (2020) in press, bbaa232, doi:10.1093/bib/bbaa232.
- Khaledi, A.*, Weimann, A.*, Schniederjans, M., Asgari, E., Kuo, T. H., Oliver, A., Cabot, G., Kola, A., Gastmeier, P., Hogardt, M., Jonas, D., Mofrad, M. R., Bremges, A., McHardy, A. C.* & Häussler, S.* (*shared first & last authors) Predicting antimicrobial resistance in Pseudomonas aeruginosa with machine learning-enabled molecular diagnostics. EMBO Mol Med (2020) 12, e10264, doi:10.15252/emmm.201910264.
- Kloetgen, A., Duggimpudi, S., Schuschel, K., Hezaveh, K., Picard, D., Schaal, H., Remke, M., Klusmann, J. H., Borkhardt, A., McHardy, A. C. & Hoell, J. I. YBX1 Indirectly Targets Heterochromatin-Repressed Inflammatory Response-Related Apoptosis Genes through Regulating CBX5 mRNA. Int J Mol Sci (2020) 21, 4453, doi:10.3390/ijms21124453.
- Kloetgen, A.*, Thandapani, P.*, Ntziachristos, P.*, Ghebrechristos, Y., Nomikou, S., Lazaris, C., Chen, X., Hu, H., Bakogianni, S., Wang, J., Fu, Y., Boccalatte, F., Zhong, H., Paietta, E., Trimarchi, T., Zhu, Y., Van Vlierberghe, P., Inghirami, G. G., Lionnet, T., Aifantis, I. & Tsirigos, A. (*shared first authors) Three-dimensional chromatin landscapes in T cell acute lymphoblastic leukemia. Nat Genet (2020) 52, 388, doi:10.1038/s41588-020-0602-9.
- Lähnemann, D., Köster, J., Szczurek, E., McCarthy, D. J., Hicks, S. C., Robinson, M. D., Vallejos, C. A., Campbell, K. R., Beerenwinkel, N., Mahfouz, A., Pinello, L., Skums, P., Stamatakis, A., Attolini, C. S., Aparicio, S., Baaijens, J., Balvert, M., Barbanson, B., Cappuccio, A., Corleone, G., Dutilh, B. E., Florescu, M., Guryev, V., Holmer, R., Jahn, K., Lobo, T. J., Keizer, E. M., Khatri, I., Kielbasa, S. M., Korbel, J. O., Kozlov, A. M., Kuo, T. H., Lelieveldt, B. P. F., Mandoiu, II, Marioni, J. C., Marschall, T., Mölder, F., Niknejad, A., Raczkowski, L., Reinders, M., Ridder, J., Saliba, A. E., Somarakis, A., Stegle, O., Theis, F. J., Yang, H., Zelikovsky, A., McHardy, A. C., Raphael, B. J., Shah, S. P. & Schönhuth, A. Eleven grand challenges in single-cell data science. Genome Biol (2020) 21, 31, doi:10.1186/s13059-020-1926-6.
- Lesker, T. R., Durairaj, A. C., Gálvez, E. J. C., Lagkouvardos, I., Baines, J. F., Clavel, T., Sczyrba, A., McHardy, A. C. & Strowig, T. An Integrated Metagenome Catalog Reveals New Insights into the Murine Gut Microbiome. Cell Rep (2020) 30, 2909, doi:10.1016/j.celrep.2020.02.036.
- Nishana, M., Ha, C., Rodriguez-Hernaez, J., Ranjbaran, A., Chio, E., Nora, E. P., Badri, S. B., Kloetgen, A., Bruneau, B. G., Tsirigos, A. & Skok, J. A. Defining the relative and combined contribution of CTCF and CTCFL to genomic regulation. Genome Biol (2020) 21, 108, doi:10.1186/s13059-020-02024-0.
- Reimering, S., Muñoz, S. & McHardy, A. C. Phylogeographic reconstruction using air transportation data and its application to the 2009 H1N1 influenza A pandemic. PLoS Comput Biol (2020) 16, e1007101, doi:10.1371/journal.pcbi.1007101.
- Rodriguez, S., Abundis, C., Boccalatte, F., Mehrotra, P., Chiang, M. Y., Yui, M. A., Wang, L., Zhang, H., Zollman, A., Bonfim-Silva, R., Kloetgen, A., Palmer, J., Sandusky, G., Wunderlich, M., Kaplan, M. H., Mulloy, J. C., Marcucci, G., Aifantis, I., Cardoso, A. A. & Carlesso, N. Therapeutic targeting of the E3 ubiquitin ligase SKP2 in T-ALL. Leukemia (2020) 34, 1241, doi:10.1038/s41375-019-0653-z.
- Schira-Heinen, J., Czapla, A., Hendricks, M., Kloetgen, A., Wruck, W., Adjaye, J., Kögler, G., Werner Müller, H., Stühler, K. & Trompeter, H. I. Functional omics analyses reveal only minor effects of microRNAs on human somatic stem cell differentiation. Sci Rep (2020) 10, 3284, doi:10.1038/s41598-020-60065-8.
- Spriewald, S., Stadler, E., Hense, B. A., Münch, P. C., McHardy, A. C., Weiss, A. S., Obeng, N., Müller, J. & Stecher, B. Evolutionary Stabilization of Cooperative Toxin Production through a Bacterium-Plasmid-Phage Interplay. mBio (2020) 11, e00912-20, doi:10.1128/mBio.00912-20.
- Thiele, S., Stanelle-Bertram, S., Beck, S., Kouassi, N. M., Zickler, M., Müller, M., Tuku, B., Resa-Infante, P., van Riel, D., Alawi, M., Günther, T., Rother, F., Hügel, S., Reimering, S., McHardy, A., Grundhoff, A., Brune, W., Osterhaus, A., Bader, M., Hartmann, E. & Gabriel, G. Cellular Importin-α3 Expression Dynamics in the Lung Regulate Antiviral Response Pathways against Influenza A Virus Infection. Cell Reports (2020) 31, 107549, doi:https://doi.org/10.1016/j.celrep.2020.107549.
2019
- Amann, R. I., Baichoo, S., Blencowe, B. J., Bork, P., Borodovsky, M., Brooksbank, C., Chain, P. S. G., Colwell, R. R., Daffonchio, D. G., Danchin, A., de Lorenzo, V., Dorrestein, P. C., Finn, R. D., Fraser, C. M., Gilbert, J. A., Hallam, S. J., Hugenholtz, P., Ioannidis, J. P. A., Jansson, J. K., Kim, J. F., Klenk, H. P., Klotz, M. G., Knight, R., Konstantinidis, K. T., Kyrpides, N. C., Mason, C. E., McHardy, A. C., Meyer, F., Ouzounis, C. A., Patrinos, A. A. N., Podar, M., Pollard, K. S., Ravel, J., Muñoz, A. R., Roberts, R. J., Rosselló-Móra, R., Sansone, S. A., Schloss, P. D., Schriml, L. M., Setubal, J. C., Sorek, R., Stevens, R. L., Tiedje, J. M., Turjanski, A., Tyson, G. W., Ussery, D. W., Weinstock, G. M., White, O., Whitman, W. B. & Xenarios, I. Consent insufficient for data release-Response. Science (2019) 364, 446, doi:10.1126/science.aax7509.
- Amann, R. I., Baichoo, S., Blencowe, B. J., Bork, P., Borodovsky, M., Brooksbank, C., Chain, P. S. G., Colwell, R. R., Daffonchio, D. G., Danchin, A., de Lorenzo, V., Dorrestein, P. C., Finn, R. D., Fraser, C. M., Gilbert, J. A., Hallam, S. J., Hugenholtz, P., Ioannidis, J. P. A., Jansson, J. K., Kim, J. F., Klenk, H. P., Klotz, M. G., Knight, R., Konstantinidis, K. T., Kyrpides, N. C., Mason, C. E., McHardy, A. C., Meyer, F., Ouzounis, C. A., Patrinos, A. A. N., Podar, M., Pollard, K. S., Ravel, J., Muñoz, A. R., Roberts, R. J., Rosselló-Móra, R., Sansone, S. A., Schloss, P. D., Schriml, L. M., Setubal, J. C., Sorek, R., Stevens, R. L., Tiedje, J. M., Turjanski, A., Tyson, G. W., Ussery, D. W., Weinstock, G. M., White, O., Whitman, W. B. & Xenarios, I. Toward unrestricted use of public genomic data. Science (2019) 363, 350, doi:10.1126/science.aaw1280.
- Asgari, E., Garakani, K., McHardy, A. C. & Mofrad, M. R. K. MicroPheno: predicting environments and host phenotypes from 16S rRNA gene sequencing using a k-mer based representation of shallow sub-samples. Bioinformatics (2019) 35, 1082, doi:10.1093/bioinformatics/bty652.
- Asgari, E., McHardy, A. C. & Mofrad, M. R. K. Probabilistic variable-length segmentation of protein sequences for discriminative motif discovery (DiMotif) and sequence embedding (ProtVecX). Sci Rep (2019) 9, 3577, doi:10.1038/s41598-019-38746-w.
- Asgari, E., Münch, P. C., Lesker, T. R., McHardy, A. C. & Mofrad, M. R. K. DiTaxa: nucleotide-pair encoding of 16S rRNA for host phenotype and biomarker detection. Bioinformatics (2019) 35, 2498, doi:10.1093/bioinformatics/bty954.
- Bledea, R., Vasudevaraja, V., Patel, S., Stafford, J., Serrano, J., Esposito, G., Tredwin, L. M., Goodman, N., Kloetgen, A., Golfinos, J. G., Zagzag, D., Weigelt, B., Iafrate, A. J., Sulman, E. P., Chi, A. S., Dogan, S., Reis-Filho, J. S., Chiang, S., Placantonakis, D., Tsirigos, A. & Snuderl, M. Functional and topographic effects on DNA methylation in IDH1/2 mutant cancers. Sci Rep (2019) 9, 16830, doi:10.1038/s41598-019-53262-7.
- Chen, X., Glytsou, C., Zhou, H., Narang, S., Reyna, D. E., Lopez, A., Sakellaropoulos, T., Gong, Y., Kloetgen, A., Yap, Y. S., Wang, E., Gavathiotis, E., Tsirigos, A., Tibes, R. & Aifantis, I. Targeting Mitochondrial Structure Sensitizes Acute Myeloid Leukemia to Venetoclax Treatment. Cancer Discov (2019) 9, 890, doi:10.1158/2159-8290.Cd-19-0117.
- Di Giammartino, D. C.*, Kloetgen, A.*, Polyzos, A., Liu, Y., Kim, D., Murphy, D., Abuhashem, A., Cavaliere, P., Aronson, B., Shah, V., Dephoure, N., Stadtfeld, M., Tsirigos, A. & Apostolou, E. (*shared first authors) KLF4 is involved in the organization and regulation of pluripotency-associated three-dimensional enhancer networks. Nat Cell Biol (2019) 21, 1179, doi:10.1038/s41556-019-0390-6.
- Fritz, A., Hofmann, P., Majda, S., Dahms, E., Dröge, J., Fiedler, J., Lesker, T. R., Belmann, P., DeMaere, M. Z., Darling, A. E., Sczyrba, A., Bremges, A. & McHardy, A. C. CAMISIM: simulating metagenomes and microbial communities. Microbiome (2019) 7, 17, doi:10.1186/s40168-019-0633-6.
- Halaby, M. J., Hezaveh, K., Lamorte, S., Ciudad, M. T., Kloetgen, A., MacLeod, B. L., Guo, M., Chakravarthy, A., Medina, T. D. S., Ugel, S., Tsirigos, A., Bronte, V., Munn, D. H., Pugh, T. J., De Carvalho, D. D., Butler, M. O., Ohashi, P. S., Brooks, D. G. & McGaha, T. L. GCN2 drives macrophage and MDSC function and immunosuppression in the tumor microenvironment. Sci Immunol (2019) 4, eaax8189, doi:10.1126/sciimmunol.aax8189.
- Hoell, J. I., Ginzel, S., Kuhlen, M., Kloetgen, A., Gombert, M., Fischer, U., Hein, D., Demir, S., Stanulla, M., Schrappe, M., Zur Stadt, U., Bader, P., Babor, F., Schuster, F., Strahm, B., Alten, J., Moericke, A., Escherich, G., von Stackelberg, A., Thiele, R., McHardy, A. C., Peters, C., Bornhauser, B., Bourquin, J. P., Krause, S., Enczmann, J., Meyer, L. H., Eckert, C., Borkhardt, A. & Meisel, R. Pediatric ALL relapses after allo-SCT show high individuality, clonal dynamics, selective pressure, and druggable targets. Blood Adv (2019) 3, 3143, doi:10.1182/bloodadvances.2019000051.
- Ingenhag, D., Reister, S., Auer, F., Bhatia, S., Wildenhain, S., Picard, D., Remke, M., Hoell, J. I., Kloetgen, A., Sohn, D., Jänicke, R. U., Koegler, G., Borkhardt, A. & Hauer, J. The homeobox transcription factor HB9 induces senescence and blocks differentiation in hematopoietic stem and progenitor cells. Haematologica (2019) 104, 35, doi:10.3324/haematol.2018.189407.
- Klingen, T. R., Loers, J., Stanelle-Bertram, S., Gabriel, G. & McHardy, A. C. Structures and functions linked to genome-wide adaptation of human influenza A viruses. Sci Rep (2019) 9, 6267, doi:10.1038/s41598-019-42614-y.
- Kloetgen, A., Münch, P. C., Borkhardt, A., Hoell, J. I. & McHardy, A. C. Corrigendum to: Biochemical and bioinformatic methods for elucidating the role of RNA-protein interactions in posttranscriptional regulation. Brief Funct Genomics (2019) 18, 433, doi:10.1093/bfgp/elz014.
- Kloetgen, A.*, Thandapani, P.*, Tsirigos, A.* & Aifantis, I.* (*shared first authors) 3D Chromosomal Landscapes in Hematopoiesis and Immunity. Trends Immunol (2019) 40, 809, doi:10.1016/j.it.2019.07.003.
- Lhoumaud, P., Badri, S., Rodriguez-Hernaez, J., Sakellaropoulos, T., Sethia, G., Kloetgen, A., Cornwell, M., Bhattacharyya, S., Ay, F., Bonneau, R., Tsirigos, A. & Skok, J. A. NSD2 overexpression drives clustered chromatin and transcriptional changes in a subset of insulated domains. Nat Commun (2019) 10, 4843, doi:10.1038/s41467-019-12811-4.
- Meyer, F., Bremges, A., Belmann, P., Janssen, S., McHardy, A. C.* & Koslicki, D.* (*shared last authors) Assessing taxonomic metagenome profilers with OPAL. Genome Biol (2019) 20, 51, doi:10.1186/s13059-019-1646-y.
- Vatanen, T., Plichta, D. R., Somani, J., Münch, P. C., Arthur, T. D., Hall, A. B., Rudolf, S., Oakeley, E. J., Ke, X., Young, R. A., Haiser, H. J., Kolde, R., Yassour, M., Luopajärvi, K., Siljander, H., Virtanen, S. M., Ilonen, J., Uibo, R., Tillmann, V., Mokurov, S., Dorshakova, N., Porter, J. A., McHardy, A. C., Lähdesmäki, H., Vlamakis, H., Huttenhower, C., Knip, M. & Xavier, R. J. Genomic variation and strain-specific functional adaptation in the human gut microbiome during early life. Nat Microbiol (2019) 4, 470, doi:10.1038/s41564-018-0321-5.
- Vatanen, T., Plichta, D. R., Somani, J., Münch, P. C., Arthur, T. D., Hall, A. B., Rudolf, S., Oakeley, E. J., Ke, X., Young, R. A., Haiser, H. J., Kolde, R., Yassour, M., Luopajärvi, K., Siljander, H., Virtanen, S. M., Ilonen, J., Uibo, R., Tillmann, V., Mokurov, S., Dorshakova, N., Porter, J. A., McHardy, A. C., Lähdesmäki, H., Vlamakis, H., Huttenhower, C., Knip, M. & Xavier, R. J. Publisher Correction: Genomic variation and strain-specific functional adaptation in the human gut microbiome during early life. Nat Microbiol (2019) 4, 545, doi:10.1038/s41564-019-0393-x.
- Wang, E., Lu, S. X., Pastore, A., Chen, X., Imig, J., Chun-Wei Lee, S., Hockemeyer, K., Ghebrechristos, Y. E., Yoshimi, A., Inoue, D., Ki, M., Cho, H., Bitner, L., Kloetgen, A., Lin, K. T., Uehara, T., Owa, T., Tibes, R., Krainer, A. R., Abdel-Wahab, O. & Aifantis, I. Targeting an RNA-Binding Protein Network in Acute Myeloid Leukemia. Cancer Cell (2019) 35, 369, doi:10.1016/j.ccell.2019.01.010.
- Zhou, N., Jiang, Y., Bergquist, T. R., Lee, A. J., Kacsoh, B. Z., Crocker, A. W., Lewis, K. A., Georghiou, G., Nguyen, H. N., Hamid, M. N., Davis, L., Dogan, T., Atalay, V., Rifaioglu, A. S., Dalkıran, A., Cetin Atalay, R., Zhang, C., Hurto, R. L., Freddolino, P. L., Zhang, Y., Bhat, P., Supek, F., Fernández, J. M., Gemovic, B., Perovic, V. R., Davidović, R. S., Sumonja, N., Veljkovic, N., Asgari, E., Mofrad, M. R. K., Profiti, G., Savojardo, C., Martelli, P. L., Casadio, R., Boecker, F., Schoof, H., Kahanda, I., Thurlby, N., McHardy, A. C., Renaux, A., Saidi, R., Gough, J., Freitas, A. A., Antczak, M., Fabris, F., Wass, M. N., Hou, J., Cheng, J., Wang, Z., Romero, A. E., Paccanaro, A., Yang, H., Goldberg, T., Zhao, C., Holm, L., Törönen, P., Medlar, A. J., Zosa, E., Borukhov, I., Novikov, I., Wilkins, A., Lichtarge, O., Chi, P. H., Tseng, W. C., Linial, M., Rose, P. W., Dessimoz, C., Vidulin, V., Dzeroski, S., Sillitoe, I., Das, S., Lees, J. G., Jones, D. T., Wan, C., Cozzetto, D., Fa, R., Torres, M., Warwick Vesztrocy, A., Rodriguez, J. M., Tress, M. L., Frasca, M., Notaro, M., Grossi, G., Petrini, A., Re, M., Valentini, G., Mesiti, M., Roche, D. B., Reeb, J., Ritchie, D. W., Aridhi, S., Alborzi, S. Z., Devignes, M. D., Koo, D. C. E., Bonneau, R., Gligorijević, V., Barot, M., Fang, H., Toppo, S., Lavezzo, E., Falda, M., Berselli, M., Tosatto, S. C. E., Carraro, M., Piovesan, D., Ur Rehman, H., Mao, Q., Zhang, S., Vucetic, S., Black, G. S., Jo, D., Suh, E., Dayton, J. B., Larsen, D. J., Omdahl, A. R., McGuffin, L. J., Brackenridge, D. A., Babbitt, P. C., Yunes, J. M., Fontana, P., Zhang, F., Zhu, S., You, R., Zhang, Z., Dai, S., Yao, S., Tian, W., Cao, R., Chandler, C., Amezola, M., Johnson, D., Chang, J. M., Liao, W. H., Liu, Y. W., Pascarelli, S., Frank, Y., Hoehndorf, R., Kulmanov, M., Boudellioua, I., Politano, G., Di Carlo, S., Benso, A., Hakala, K., Ginter, F., Mehryary, F., Kaewphan, S., Björne, J., Moen, H., Tolvanen, M. E. E., Salakoski, T., Kihara, D., Jain, A., Šmuc, T., Altenhoff, A., Ben-Hur, A., Rost, B., Brenner, S. E., Orengo, C. A., Jeffery, C. J., Bosco, G., Hogan, D. A., Martin, M. J., O'Donovan, C., Mooney, S. D., Greene, C. S., Radivojac, P.* & Friedberg, I.* (*shared last authors) The CAFA challenge reports improved protein function prediction and new functional annotations for hundreds of genes through experimental screens. Genome Biol (2019) 20, 244, doi:10.1186/s13059-019-1835-8.
2018
- Asgari, E., Garakani, K., McHardy, A. C.* & Mofrad, M. R. K.* (*shared last authors) MicroPheno: predicting environments and host phenotypes from 16S rRNA gene sequencing using a k-mer based representation of shallow sub-samples. Bioinformatics (2018) 34, i32, doi:10.1093/bioinformatics/bty296.
- Bremges, A. & McHardy, A. C. Critical Assessment of Metagenome Interpretation Enters the Second Round. mSystems (2018) 3, e00103-18, doi:10.1128/mSystems.00103-18.
- Duggimpudi, S., Kloetgen, A., Maney, S. K., Münch, P. C., Hezaveh, K., Shaykhalishahi, H., Hoyer, W., McHardy, A. C., Lang, P. A., Borkhardt, A. & Hoell, J. I. Transcriptome-wide analysis uncovers the targets of the RNA-binding protein MSI2 and effects of MSI2's RNA-binding activity on IL-6 signaling. J Biol Chem (2018) 293, 15359, doi:10.1074/jbc.RA118.002243.
- Garrido-Oter, R., Nakano, R. T., Dombrowski, N., Ma, K. W., McHardy, A. C. & Schulze-Lefert, P. Modular Traits of the Rhizobiales Root Microbiota and Their Evolutionary Relationship with Symbiotic Rhizobia. Cell Host Microbe (2018) 24, 155, doi:10.1016/j.chom.2018.06.006.
- Hoell, J. I., Ginzel, S., Kuhlen, M., Klötgen, A., Gombert, M., Fischer, U., Schäfer, D., Stanulla, M., Schrappe, M., Stadt, U. z., Bader, P., Babor, F., Schuster, F., Strahm, B., Alten, J., Moericke, A., Escherich, G., von Stackelberg, A., Thiele, R., McHardy, A. C., Peters, C., Eckert, C., Borkhardt, A. & Meisel, R. Whole-Exome-Sequencing identifiziert hohe Plastizität, Selektionsdruck und „druggable targets“ in Leukämiezellen pädiatrischer ALL Rezidive nach allogener Stammzelltransplantation. Monatsschrift Kinderheilkunde (2018) 166, 648.
- Ibrahim, B., Arkhipova, K., Andeweg, A. C., Posada-Céspedes, S., Enault, F., Gruber, A., Koonin, E. V., Kupczok, A., Lemey, P., McHardy, A. C., McMahon, D. P., Pickett, B. E., Robertson, D. L., Scheuermann, R. H., Zhernakova, A., Zwart, M. P., Schönhuth, A., Dutilh, B. E. & Marz, M. Bioinformatics Meets Virology: The European Virus Bioinformatics Center's Second Annual Meeting. Viruses (2018) 10, 256, doi:10.3390/v10050256.
- Klingen, T. R.*, Reimering, S.*, Guzmán, C. A. & McHardy, A. C. (*shared first authors) In Silico Vaccine Strain Prediction for Human Influenza Viruses. Trends Microbiol (2018) 26, 119, doi:10.1016/j.tim.2017.09.001.
- Klingen, T. R.*, Reimering, S.*, Loers, J., Mooren, K., Klawonn, F., Krey, T., Gabriel, G. & McHardy, A. C. (*shared first authors) Sweep Dynamics (SD) plots: Computational identification of selective sweeps to monitor the adaptation of influenza A viruses. Sci Rep (2018) 8, 373, doi:10.1038/s41598-017-18791-z.
- Meyer, F., Hofmann, P., Belmann, P., Garrido-Oter, R., Fritz, A., Sczyrba, A. & McHardy, A. C. AMBER: Assessment of Metagenome BinnERs. Gigascience (2018) 7, giy069, doi:10.1093/gigascience/giy069.
- Naas, A. E., Solden, L. M., Norbeck, A. D., Brewer, H., Hagen, L. H., Heggenes, I. M., McHardy, A. C., Mackie, R. I., Paša-Tolić, L., Arntzen, M., Eijsink, V. G. H., Koropatkin, N. M., Hess, M., Wrighton, K. C. & Pope, P. B. "Candidatus Paraporphyromonas polyenzymogenes" encodes multi-modular cellulases linked to the type IX secretion system. Microbiome (2018) 6, 44, doi:10.1186/s40168-018-0421-8.
- Reimering, S. & McHardy, A. C. Software for Characterizing the Antigenic and Genetic Evolution of Human Influenza Viruses. Methods Mol Biol (2018) 1836, 551, doi:10.1007/978-1-4939-8678-1_26.
- Reimering, S., Muñoz, S. & McHardy, A. C. A Fréchet tree distance measure to compare phylogeographic spread paths across trees. Sci Rep (2018) 8, 17000, doi:10.1038/s41598-018-35421-4.
2017
- Doerrenberg, M., Kloetgen, A., Hezaveh, K., Wossmann, W., Bleckmann, K., Stanulla, M., Schrappe, M., McHardy, A. C., Borkhardt, A. & Hoell, J. I. T-cell acute lymphoblastic leukemia in infants has distinct genetic and epigenetic features compared to childhood cases. Genes Chromosomes Cancer (2017) 56, 159, doi:10.1002/gcc.22423.
- Dröge, J., Schonhuth, A. & McHardy, A. C. A probabilistic model to recover individual genomes from metagenomes. PeerJ Computer Science (2017) 3, e117.
- Grießmeier, V., Bremges, A., McHardy, A. C. & Gescher, J. Investigation of different nitrogen reduction routes and their key microbial players in wood chip-driven denitrification beds. Scientific Reports (2017) 7, 17028, doi:10.1038/s41598-017-17312-2.
- Krause, S., Bremges, A., Munch, P. C., McHardy, A. C. & Gescher, J. Characterisation of a stable laboratory co-culture of acidophilic nanoorganisms. Sci Rep (2017) 7, 3289, doi:10.1038/s41598-017-03315-6.
- Kunath, B. J., Bremges, A., Weimann, A., McHardy, A. C. & Pope, P. B. Metagenomics and CAZyme Discovery. Methods Mol Biol (2017) 1588, 255, doi:10.1007/978-1-4939-6899-2_20.
- Maus, I.*, Bremges, A.*, Stolze, Y., Hahnke, S., Cibis, K. G., Koeck, D. E., Kim, Y. S., Kreubel, J., Hassa, J., Wibberg, D., Weimann, A., Off, S., Stantscheff, R., Zverlov, V. V., Schwarz, W. H., König, H., Liebl, W., Scherer, P., McHardy, A. C., Sczyrba, A., Klocke, M., Pühler, A. & Schlüter, A. (*shared first authors) Genomics and prevalence of bacterial and archaeal isolates from biogas-producing microbiomes. Biotechnology for Biofuels (2017) 10, 264, doi:10.1186/s13068-017-0947-1.
- McHardy, A. C. & Kloetgen, A. Finding Genes in Genome Sequence. Methods Mol Biol (2017) 1525, 271, doi:10.1007/978-1-4939-6622-6_11.
- Münch, P. C., Stecher, B. & McHardy, A. C. EDEN: Evolutionary Dynamics within Environments. Bioinformatics (2017), 33, 3292 doi:10.1093/bioinformatics/btx394.
- Sczyrba, A.*, Hofmann, P.*, Belmann, P.*, Koslicki, D., Janssen, S., Dröge, J., Gregor, I., Majda, S., Fiedler, J., Dahms, E., Bremges, A., Fritz, A., Garrido-Oter, R., Jørgensen, T. S., Shapiro, N., Blood, P. D., Gurevich, A., Bai, Y., Turaev, D., DeMaere, M. Z., Chikhi, R., Nagarajan, N., Quince, C., Meyer, F., Balvočiūtė, M., Hansen, L. H., Sørensen, S. J., Chia, B. K. H., Denis, B., Froula, J. L., Wang, Z., Egan, R., Don Kang, D., Cook, J. J., Deltel, C., Beckstette, M., Lemaitre, C., Peterlongo, P., Rizk, G., Lavenier, D., Wu, Y.-W., Singer, S. W., Jain, C., Strous, M., Klingenberg, H., Meinicke, P., Barton, M. D., Lingner, T., Lin, H.-H., Liao, Y.-C., Silva, G. G. Z., Cuevas, D. A., Edwards, R. A., Saha, S., Piro, V. C., Renard, B. Y., Pop, M., Klenk, H.-P., Göker, M., Kyrpides, N. C., Woyke, T., Vorholt, J. A., Schulze-Lefert, P., Rubin, E. M., Darling, A. E., Rattei, T. & McHardy, A. C. (*shared first authors) Critical Assessment of Metagenome Interpretation—a benchmark of metagenomics software. Nature Methods (2017) 14, 1063, doi:10.1038/nmeth.4458
- Ye, Z., Pan, Y., Zhang, Y., Cui, H., Jin, G., McHardy, A. C., Fan, L. & Yu, X. Comparative whole-genome analysis reveals artificial selection effects on Ustilago esculenta genome. DNA Research (2017), 24, 635, doi:10.1093/dnares/dsx031.
2016
- Brugiroux, S., Beutler, M., Pfann, C., Garzetti, D., Ruscheweyh, H. J., Ring, D., Diehl, M., Herp, S., Lotscher, Y., Hussain, S., Bunk, B., Pukall, R., Huson, D. H., Munch, P. C., McHardy, A. C., McCoy, K. D., Macpherson, A. J., Loy, A., Clavel, T., Berry, D. & Stecher, B. Genome-guided design of a defined mouse microbiota that confers colonization resistance against Salmonella enterica serovar Typhimurium. Nat Microbiol (2016) 2, 16215, doi:10.1038/nmicrobiol.2016.215.
- Dong, X., Droge, J., von Toerne, C., Marozava, S., McHardy, A. C. & Meckenstock, R. U. Reconstructing metabolic pathways of a member of the genus Pelotomaculum suggesting its potential to oxidize benzene to carbon dioxide with direct reduction of sulfate. FEMS Microbiol Ecol (2016), 93, doi:10.1093/femsec/fiw254.
- Frank, J. A., Arntzen, M. O., Sun, L., Hagen, L. H., McHardy, A. C., Horn, S. J., Eijsink, V. G., Schnurer, A. & Pope, P. B. Novel Syntrophic Populations Dominate an Ammonia-Tolerant Methanogenic Microbiome. mSystems (2016) 1, doi:10.1128/mSystems.00092-16.
- Frank, J. A., Pan, Y., Tooming-Klunderud, A., Eijsink, V. G., McHardy, A. C., Nederbragt, A. J. & Pope, P. B. Improved metagenome assemblies and taxonomic binning using long-read circular consensus sequence data. Sci Rep (2016) 6, 25373, doi:10.1038/srep25373.
- Gregor, I., Droge, J., Schirmer, M., Quince, C. & McHardy, A. C. PhyloPythiaS+: a self-training method for the rapid reconstruction of low-ranking taxonomic bins from metagenomes. PeerJ (2016) 4, e1603, doi:10.7717/peerj.1603.
- Gregor, I., Schonhuth, A. & McHardy, A. C. Snowball: strain aware gene assembly of metagenomes. Bioinformatics (2016) 32, i649, doi:10.1093/bioinformatics/btw426.
- Hacquard, S., Kracher, B., Hiruma, K., Munch, P. C., Garrido-Oter, R., Thon, M. R., Weimann, A., Damm, U., Dallery, J. F., Hainaut, M., Henrissat, B., Lespinet, O., Sacristan, S., Ver Loren van Themaat, E., Kemen, E., McHardy, A. C., Schulze-Lefert, P. & O'Connell, R. J. Survival trade-offs in plant roots during colonization by closely related beneficial and pathogenic fungi. Nat Commun (2016) 7, 11362, doi:10.1038/ncomms11362.
- Hezaveh, K., Kloetgen, A., Bernhart, S. H., Mahapatra, K. D., Lenze, D., Richter, J., Haake, A., Bergmann, A. K., Brors, B., Burkhardt, B., Claviez, A., Drexler, H. G., Eils, R., Haas, S., Hoffmann, S., Karsch, D., Klapper, W., Kleinheinz, K., Korbel, J., Kretzmer, H., Kreuz, M., Kuppers, R., Lawerenz, C., Leich, E., Loeffler, M., Mantovani-Loeffler, L., Lopez, C., McHardy, A. C., Moller, P., Rohde, M., Rosenstiel, P., Rosenwald, A., Schilhabel, M., Schlesner, M., Scholz, I., Stadler, P. F., Stilgenbauer, S., Sungalee, S., Szczepanowski, M., Trumper, L., Weniger, M. A., Siebert, R., Borkhardt, A., Hummel, M. & Hoell, J. I. Alterations of miRNAs and miRNA-regulated mRNA expression in GC B cell lymphomas determined by integrative sequencing analysis. Haematologica (2016), doi:10.3324/haematol.2016.143891.
- Ikeda-Ohtsubo, W., Strassert, J. F., Kohler, T., Mikaelyan, A., Gregor, I., McHardy, A. C., Tringe, S. G., Hugenholtz, P., Radek, R. & Brune, A. 'Candidatus Adiutrix intracellularis', an endosymbiont of termite gut flagellates, is the first representative of a deep-branching clade of Deltaproteobacteria and a putative homoacetogen. Environ Microbiol (2016) 18, 2548, doi:10.1111/1462-2920.13234.
- Kloetgen, A., Borkhardt, A., Hoell, J. I. & McHardy, A. C. The PARA-suite: PAR-CLIP specific sequence read simulation and processing. PeerJ (2016) 4, e2619, doi:10.7717/peerj.2619.
- Kratsch, C.*, Klingen, T. R.*, Mumken, L., Steinbruck, L. & McHardy, A. C. (*shared first authors) Determination of antigenicity-altering patches on the major surface protein of human influenza A/H3N2 viruses. Virus Evol (2016) 2, vev025, doi:10.1093/ve/vev025.
- Laehnemann, D., Borkhardt, A. & McHardy, A. C. Denoising DNA deep sequencing data-high-throughput sequencing errors and their correction. Brief Bioinform (2016) 17, 154, doi:10.1093/bib/bbv029.
- Otte, A., Marriott, A. C., Dreier, C., Dove, B., Mooren, K., Klingen, T. R., Sauter, M., Thompson, K. A., Bennett, A., Klingel, K., van Riel, D., McHardy, A. C., Carroll, M. W. & Gabriel, G. Evolution of 2009 H1N1 influenza viruses during the pandemic correlates with increased viral pathogenicity and transmissibility in the ferret model. Sci Rep (2016) 6, 28583, doi:10.1038/srep28583.
- Weimann, A., Mooren, K., Frank, J., Pope, P. B., Bremges, A. & McHardy, A. C. From Genomes to Phenotypes: Traitar, the Microbial Trait Analyzer. mSystems (2016) 1, doi:10.1128/mSystems.00101-16.
2015
- Bai, Y., Muller, D. B., Srinivas, G., Garrido-Oter, R., Potthoff, E., Rott, M., Dombrowski, N., Munch, P. C., Spaepen, S., Remus-Emsermann, M., Huttel, B., McHardy, A. C., Vorholt, J. A. & Schulze-Lefert, P. Functional overlap of the Arabidopsis leaf and root microbiota. Nature (2015) 528, 364, doi:10.1038/nature16192.
- Belmann, P., Droge, J., Bremges, A., McHardy, A. C., Sczyrba, A. & Barton, M. D. Bioboxes: standardised containers for interchangeable bioinformatics software. Gigascience (2015) 4, 47, doi:10.1186/s13742-015-0087-0.
- Bulgarelli, D.*, Garrido-Oter, R.*, Munch, P. C., Weiman, A., Droge, J., Pan, Y., McHardy, A. C.* & Schulze-Lefert, P.* (*shared first & last authors) Structure and function of the bacterial root microbiota in wild and domesticated barley. Cell Host Microbe (2015) 17, 392, doi:10.1016/j.chom.2015.01.011.
- Droge, J., Gregor, I. & McHardy, A. C. Taxator-tk: precise taxonomic assignment of metagenomes by fast approximation of evolutionary neighborhoods. Bioinformatics (2015) 31, 817, doi:10.1093/bioinformatics/btu745.
- Fischer, U., Forster, M., Rinaldi, A., Risch, T., Sungalee, S., Warnatz, H. J., Bornhauser, B., Gombert, M., Kratsch, C., Stutz, A. M., Sultan, M., Tchinda, J., Worth, C. L., Amstislavskiy, V., Badarinarayan, N., Baruchel, A., Bartram, T., Basso, G., Canpolat, C., Cario, G., Cave, H., Dakaj, D., Delorenzi, M., Dobay, M. P., Eckert, C., Ellinghaus, E., Eugster, S., Frismantas, V., Ginzel, S., Haas, O. A., Heidenreich, O., Hemmrich-Stanisak, G., Hezaveh, K., Holl, J. I., Hornhardt, S., Husemann, P., Kachroo, P., Kratz, C. P., Kronnie, G. T., Marovca, B., Niggli, F., McHardy, A. C., Moorman, A. V., Panzer-Grumayer, R., Petersen, B. S., Raeder, B., Ralser, M., Rosenstiel, P., Schafer, D., Schrappe, M., Schreiber, S., Schutte, M., Stade, B., Thiele, R., Weid, N., Vora, A., Zaliova, M., Zhang, L., Zichner, T., Zimmermann, M., Lehrach, H., Borkhardt, A., Bourquin, J. P., Franke, A., Korbel, J. O., Stanulla, M. & Yaspo, M. L. Genomics and drug profiling of fatal TCF3-HLF-positive acute lymphoblastic leukemia identifies recurrent mutation patterns and therapeutic options. Nat Genet (2015) 47, 1020, doi:10.1038/ng.3362.
- Gubry-Rangin, C., Kratsch, C., Williams, T. A., McHardy, A. C., Embley, T. M., Prosser, J. I. & Macqueen, D. J. Coupling of diversification and pH adaptation during the evolution of terrestrial Thaumarchaeota. Proc Natl Acad Sci U S A (2015) 112, 9370, doi:10.1073/pnas.1419329112.
- Hacquard, S., Garrido-Oter, R., Gonzalez, A., Spaepen, S., Ackermann, G., Lebeis, S., McHardy, A. C., Dangl, J. L., Knight, R., Ley, R. & Schulze-Lefert, P. Microbiota and Host Nutrition across Plant and Animal Kingdoms. Cell Host Microbe (2015) 17, 603, doi:10.1016/j.chom.2015.04.009.
- Kloetgen, A., Munch, P. C., Borkhardt, A., Hoell, J. I. & McHardy, A. C. Biochemical and bioinformatic methods for elucidating the role of RNA-protein interactions in posttranscriptional regulation. Brief Funct Genomics (2015) 14, 102, doi:10.1093/bfgp/elu020.
- McHardy, A. C. How to Grow a Computational Biology Lab. PLoS Comput Biol (2015) 11, e1004397, doi:10.1371/journal.pcbi.1004397.
- McHardy, A. C. PhyloPythia(S). In: Encyclopedia of Metagenomics - Genes, Genomes and Metagenomes. Basics, Methods, Databases and Tools, Karen E. Nelson (Editor), Springer US, 2015, 582.
- Otte, A., Sauter, M., Daxer, M. A., McHardy, A. C., Klingel, K. & Gabriel, G. Adaptive Mutations That Occurred during Circulation in Humans of H1N1 Influenza Virus in the 2009 Pandemic Enhance Virulence in Mice. J Virol (2015) 89, 7329, doi:10.1128/JVI.00665-15.
2014
- Guttman, D. S.*, McHardy, A. C.* & Schulze-Lefert, P.* (*all authors contributed equally) Microbial genome-enabled insights into plant-microorganism interactions. Nature reviews. Genetics (2014) 15, 797, doi:10.1038/nrg3748.
- Konietzny, S. G., Pope, P. B., Weimann, A. & McHardy, A. C. Inference of phenotype-defining functional modules of protein families for microbial plant biomass degraders. Biotechnol Biofuels (2014) 7, 124, doi:10.1186/s13068-014-0124-8.
- Kratsch, C. & McHardy, A. C. RidgeRace: ridge regression for continuous ancestral character estimation on phylogenetic trees. Bioinformatics (2014) 30, i527, doi:10.1093/bioinformatics/btu477.
- Steinbruck, L., Klingen, T. R. & McHardy, A. C. Computational prediction of vaccine strains for human Influenza A (H3N2) viruses. J Virol (2014) 88, 12123, doi:10.1128/JVI.01861-14.
2013
- Gregor, I., Steinbruck, L. & McHardy, A. C. PTree: pattern-based, stochastic search for maximum parsimony phylogenies. PeerJ (2013) 1, e89, doi:10.7717/peerj.89.
- Patil, K. R. & McHardy, A. C. Alignment-free genome tree inference by learning group-specific distance metrics. Genome Biol Evol (2013) 5, 1470, doi:10.1093/gbe/evt105.
- Rohr, C., Kerick, M., Fischer, A., Kuhn, A., Kashofer, K., Timmermann, B., Daskalaki, A., Meinel, T., Drichel, D., Borno, S. T., Nowka, A., Krobitsch, S., McHardy, A. C., Kratsch, C., Becker, T., Wunderlich, A., Barmeyer, C., Viertler, C., Zatloukal, K., Wierling, C., Lehrach, H. & Schweiger, M. R. High-throughput miRNA and mRNA sequencing of paired colorectal normal, tumor and metastasis tissues and bioinformatic modeling of miRNA-1 therapeutic applications. PLoS One (2013) 8, e67461, doi:10.1371/journal.pone.0067461.
- Steinbrück, L. & Mchardy, A. C. Evaluating the Evolutionary Dynamics of Viral Populations. In: Dynamic Models of Infectious Diseases, V. Sree, Hari Rao (Editors), Springer, 2013, 205, doi:10.1007/978-1-4614-9224-5_8.
- Weimann, A.*, Trukhina, Y.*, Pope, P. B., Konietzny, S. G. & McHardy, A. C. (*shared first authors) De novo prediction of the genomic components and capabilities for microbial plant biomass degradation from (meta-)genomes. Biotechnol Biofuels (2013) 6, 24, doi:10.1186/1754-6834-6-24.
2012
- Droge, J. & McHardy, A. C. Taxonomic binning of metagenome samples generated by next-generation sequencing technologies. Brief Bioinform (2012) 13, 646, doi:10.1093/bib/bbs031.
- Gilbert, J. A., Steele, J. A., Caporaso, J. G., Steinbruck, L., Reeder, J., Temperton, B., Huse, S., McHardy, A. C., Knight, R., Joint, I., Somerfield, P., Fuhrman, J. A. & Field, D. Defining seasonal marine microbial community dynamics. Isme J (2012) 6, 298, doi:10.1038/ismej.2011.107.
- Patil, K. R., Roune, L. & McHardy, A. C. The PhyloPythiaS web server for taxonomic assignment of metagenome sequences. PLoS One (2012) 7, e38581, doi:10.1371/journal.pone.0038581.
- Pope, P. B., Mackenzie, A. K., Gregor, I., Smith, W., Sundset, M. A., McHardy, A. C., Morrison, M. & Eijsink, V. G. Metagenomics of the Svalbard reindeer rumen microbiome reveals abundance of polysaccharide utilization loci. PLoS One (2012) 7, e38571, doi:10.1371/journal.pone.0038571.
- Steinbruck, L. & McHardy, A. C. Inference of genotype-phenotype relationships in the antigenic evolution of human influenza A (H3N2) viruses. PLoS Comput Biol (2012) 8, e1002492, doi:10.1371/journal.pcbi.1002492.
- Tusche, C., Steinbruck, L. & McHardy, A. C. Detecting patches of protein sites of influenza A viruses under positive selection. Mol Biol Evol (2012) 29, 2063, doi:10.1093/molbev/mss095.
2011
- Adams, B. & McHardy, A. C. The impact of seasonal and year-round transmission regimes on the evolution of influenza A virus. Proc Biol Sci (2011) 278, 2249, doi:10.1098/rspb.2010.2191.
- Konietzny, S. G., Dietz, L. & McHardy, A. C. Inferring functional modules of protein families with probabilistic topic models. BMC Bioinformatics (2011) 12, 141, doi:10.1186/1471-2105-12-141.
- Lykidis, A., Chen, C. L., Tringe, S. G., McHardy, A. C., Copeland, A., Kyrpides, N. C., Hugenholtz, P., Macarie, H., Olmos, A., Monroy, O. & Liu, W. T. Multiple syntrophic interactions in a terephthalate-degrading methanogenic consortium. Isme J (2011) 5, 122, doi:10.1038/ismej.2010.125.
- McHardy, A. C. & Patil, K. R. Phylogenetic Binning of Metagenome Sequence Samples. In: Handbook of Molecular Microbial Ecology I: Metagenomics and Complementary, Frans J. de Bruijn (Editor), John Wiley & Sons, 2011, 353.
- Patil, K. R., Haider, P., Pope, P. B., Turnbaugh, P. J., Morrison, M., Scheffer, T. & McHardy, A. C. Taxonomic metagenome sequence assignment with structured output models. Nat Methods (2011) 8, 191, doi:10.1038/nmeth0311-191.
- Pope, P. B., Smith, W., Denman, S. E., Tringe, S. G., Barry, K., Hugenholtz, P., McSweeney, C. S., McHardy, A. C. & Morrison, M. Isolation of Succinivibrionaceae implicated in low methane emissions from Tammar wallabies. Science (2011) 333, 646, doi:10.1126/science.1205760.
- Steinbruck, L. & McHardy, A. C. Allele dynamics plots for the study of evolutionary dynamics in viral populations. Nucleic Acids Res (2011) 39, e4, doi:10.1093/nar/gkq909.
2010
- Gilbert, J. A., Meyer, F., Antonopoulos, D., Balaji, P., Brown, C. T., Brown, C. T., Desai, N., Eisen, J. A., Evers, D., Field, D., Feng, W., Huson, D., Jansson, J., Knight, R., Knight, J., Kolker, E., Konstantindis, K., Kostka, J., Kyrpides, N., Mackelprang, R., McHardy, A., Quince, C., Raes, J., Sczyrba, A., Shade, A. & Stevens, R. Meeting report: the terabase metagenomics workshop and the vision of an Earth microbiome project. Standards in genomic sciences (2010) 3, 243, doi:10.4056/sigs.1433550.
- Pope, P. B., Denman, S. E., Jones, M., Tringe, S. G., Barry, K., Malfatti, S. A., McHardy, A. C., Cheng, J.-F., Hugenholtz, P., McSweeney, C. S. & Morrison, M. Adaptation to herbivory by the Tammar wallaby includes bacterial and glycoside hydrolase profiles different from other herbivores. Proceedings of the National Academy of Sciences (2010) 107, 14793, doi:10.1073/pnas.1005297107.
- Turnbaugh, P. J., Quince, C., Faith, J. J., McHardy, A. C., Yatsunenko, T., Niazi, F., Affourtit, J., Egholm, M., Henrissat, B., Knight, R. & Gordon, J. I. Organismal, genetic, and transcriptional variation in the deeply sequenced gut microbiomes of identical twins. Proceedings of the National Academy of Sciences (2010) 107, 7503, doi:10.1073/pnas.1002355107.
2009
- McHardy, A. C. & Adams, B. The role of genomics in tracking the evolution of influenza A virus. PLoS Pathog (2009) 5, e1000566, doi:10.1371/journal.ppat.1000566.
2008
- Adams, B., McHardy, A. C., Lundegard, C. & Lengauer, T. Viral Bioinformatics. In: Modern Genome Annotation, A. Valencia, D. Frishman (Editors), Springer, 2008, 429.
- Gartemann, K. H., Abt, B., Bekel, T., Burger, A., Engemann, J., Flugel, M., Gaigalat, L., Goesmann, A., Grafen, I., Kalinowski, J., Kaup, O., Kirchner, O., Krause, L., Linke, B., McHardy, A. C., Meyer, F., Pohle, S., Ruckert, C., Schneiker, S., Zellermann, E. M., Puhler, A., Eichenlaub, R., Kaiser, O. & Bartels, D. The genome sequence of the tomato-pathogenic actinomycete Clavibacter michiganensis subsp. michiganensis NCPPB382 reveals a large island involved in pathogenicity. J Bacteriol (2008) 190, 2138, doi:10.1128/JB.01595-07.
- Holland, L. Z., Albalat, R., Azumi, K., Benito-Gutierrez, E., Blow, M. J., Bronner-Fraser, M., Brunet, F., Butts, T., Candiani, S., Dishaw, L. J., Ferrier, D. E., Garcia-Fernandez, J., Gibson-Brown, J. J., Gissi, C., Godzik, A., Hallbook, F., Hirose, D., Hosomichi, K., Ikuta, T., Inoko, H., Kasahara, M., Kasamatsu, J., Kawashima, T., Kimura, A., Kobayashi, M., Kozmik, Z., Kubokawa, K., Laudet, V., Litman, G. W., McHardy, A. C., Meulemans, D., Nonaka, M., Olinski, R. P., Pancer, Z., Pennacchio, L. A., Pestarino, M., Rast, J. P., Rigoutsos, I., Robinson-Rechavi, M., Roch, G., Saiga, H., Sasakura, Y., Satake, M., Satou, Y., Schubert, M., Sherwood, N., Shiina, T., Takatori, N., Tello, J., Vopalensky, P., Wada, S., Xu, A., Ye, Y., Yoshida, K., Yoshizaki, F., Yu, J. K., Zhang, Q., Zmasek, C. M., de Jong, P. J., Osoegawa, K., Putnam, N. H., Rokhsar, D. S., Satoh, N. & Holland, P. W. The amphioxus genome illuminates vertebrate origins and cephalochordate biology. Genome Res (2008) 18, 1100, doi:10.1101/gr.073676.107.
- Kalyuzhnaya, M. G., Lapidus, A., Ivanova, N., Copeland, A. C., McHardy, A. C., Szeto, E., Salamov, A., Grigoriev, I. V., Suciu, D., Levine, S. R., Markowitz, V. M., Rigoutsos, I., Tringe, S. G., Bruce, D. C., Richardson, P. M., Lidstrom, M. E. & Chistoserdova, L. High-resolution metagenomics targets specific functional types in complex microbial communities. Nat Biotechnol (2008) 26, 1029, doi:10.1038/nbt.1488.
- McHardy, A. C. Finding genes in genome sequence. Methods Mol Biol (2008) 452, 163, doi:10.1007/978-1-60327-159-2_8.
2007
- Krause, L.*, McHardy, A. C.*, Nattkemper, T. W., Puhler, A., Stoye, J. & Meyer, F. (*shared first authors) GISMO--gene identification using a support vector machine for ORF classification. Nucleic Acids Res (2007) 35, 540, doi:10.1093/nar/gkl1083.
- Mavromatis, K., Ivanova, N., Barry, K., Shapiro, H., Goltsman, E., McHardy, A. C., Rigoutsos, I., Salamov, A., Korzeniewski, F., Land, M., Lapidus, A., Grigoriev, I., Richardson, P., Hugenholtz, P. & Kyrpides, N. C. Use of simulated data sets to evaluate the fidelity of metagenomic processing methods. Nat Methods (2007) 4, 495, doi:10.1038/nmeth1043.
- McHardy, A. C., Martin, H. G., Tsirigos, A., Hugenholtz, P. & Rigoutsos, I. Accurate phylogenetic classification of variable-length DNA fragments. Nat Methods (2007) 4, 63, doi:10.1038/nmeth976.
- McHardy, A. C. & Rigoutsos, I. What's in the mix: phylogenetic classification of metagenome sequence samples. Curr Opin Microbiol (2007) 10, 499, doi:10.1016/j.mib.2007.08.004.
- Schneiker, S., Perlova, O., Kaiser, O., Gerth, K., Alici, A., Altmeyer, M. O., Bartels, D., Bekel, T., Beyer, S., Bode, E., Bode, H. B., Bolten, C. J., Choudhuri, J. V., Doss, S., Elnakady, Y. A., Frank, B., Gaigalat, L., Goesmann, A., Groeger, C., Gross, F., Jelsbak, L., Jelsbak, L., Kalinowski, J., Kegler, C., Knauber, T., Konietzny, S., Kopp, M., Krause, L., Krug, D., Linke, B., Mahmud, T., Martinez-Arias, R., McHardy, A. C., Merai, M., Meyer, F., Mormann, S., Munoz-Dorado, J., Perez, J., Pradella, S., Rachid, S., Raddatz, G., Rosenau, F., Ruckert, C., Sasse, F., Scharfe, M., Schuster, S. C., Suen, G., Treuner-Lange, A., Velicer, G. J., Vorholter, F. J., Weissman, K. J., Welch, R. D., Wenzel, S. C., Whitworth, D. E., Wilhelm, S., Wittmann, C., Blocker, H., Puhler, A. & Muller, R. Complete genome sequence of the myxobacterium Sorangium cellulosum. Nat Biotechnol (2007) 25, 1281, doi:10.1038/nbt1354.
- Warnecke, F., Luginbuhl, P., Ivanova, N., Ghassemian, M., Richardson, T. H., Stege, J. T., Cayouette, M., McHardy, A. C., Djordjevic, G., Aboushadi, N., Sorek, R., Tringe, S. G., Podar, M., Martin, H. G., Kunin, V., Dalevi, D., Madejska, J., Kirton, E., Platt, D., Szeto, E., Salamov, A., Barry, K., Mikhailova, N., Kyrpides, N. C., Matson, E. G., Ottesen, E. A., Zhang, X., Hernandez, M., Murillo, C., Acosta, L. G., Rigoutsos, I., Tamayo, G., Green, B. D., Chang, C., Rubin, E. M., Mathur, E. J., Robertson, D. E., Hugenholtz, P. & Leadbetter, J. R. Metagenomic and functional analysis of hindgut microbiota of a wood-feeding higher termite. Nature (2007) 450, 560, doi:10.1038/nature06269.
2006
- Garcia Martin, H., Ivanova, N., Kunin, V., Warnecke, F., Barry, K. W., McHardy, A. C., Yeates, C., He, S., Salamov, A. A., Szeto, E., Dalin, E., Putnam, N. H., Shapiro, H. J., Pangilinan, J. L., Rigoutsos, I., Kyrpides, N. C., Blackall, L. L., McMahon, K. D. & Hugenholtz, P. Metagenomic analysis of two enhanced biological phosphorus removal (EBPR) sludge communities. Nat Biotechnol (2006) 24, 1263, doi:10.1038/nbt1247.
- Krause, A., Ramakumar, A., Bartels, D., Battistoni, F., Bekel, T., Boch, J., Bohm, M., Friedrich, F., Hurek, T., Krause, L., Linke, B., McHardy, A. C., Sarkar, A., Schneiker, S., Syed, A. A., Thauer, R., Vorholter, F. J., Weidner, S., Puhler, A., Reinhold-Hurek, B., Kaiser, O. & Goesmann, A. Complete genome of the mutualistic, N2-fixing grass endophyte Azoarcus sp. strain BH72. Nat Biotechnol (2006) 24, 1385, doi:10.1038/nbt1243.
- Linke, B.*, McHardy, A. C.*, Neuweger, H., Krause, L. & Meyer, F. (*shared first authors) REGANOR: a gene prediction server for prokaryotic genomes and a database of high quality gene predictions for prokaryotes. Appl Bioinformatics (2006) 5, 193, doi:10.2165/00822942-200605030-00008
- Rigoutsos, I., Huynh, T., Miranda, K., Tsirigos, A., McHardy, A. C. & Platt, D. Short blocks from the noncoding parts of the human genome have instances within nearly all known genes and relate to biological processes. Proc Natl Acad Sci U S A (2006) 103, 6605, doi:10.1073/pnas.0601688103.
- Schneiker, S., Martins dos Santos, V. A., Bartels, D., Bekel, T., Brecht, M., Buhrmester, J., Chernikova, T. N., Denaro, R., Ferrer, M., Gertler, C., Goesmann, A., Golyshina, O. V., Kaminski, F., Khachane, A. N., Lang, S., Linke, B., McHardy, A. C., Meyer, F., Nechitaylo, T., Puhler, A., Regenhardt, D., Rupp, O., Sabirova, J. S., Selbitschka, W., Yakimov, M. M., Timmis, K. N., Vorholter, F. J., Weidner, S., Kaiser, O. & Golyshin, P. N. Genome sequence of the ubiquitous hydrocarbon-degrading marine bacterium Alcanivorax borkumensis. Nat Biotechnol (2006) 24, 997, doi:10.1038/nbt1232.
2005
- Overbeek, R., Begley, T., Butler, R. M., Choudhuri, J. V., Chuang, H. Y., Cohoon, M., de Crecy-Lagard, V., Diaz, N., Disz, T., Edwards, R., Fonstein, M., Frank, E. D., Gerdes, S., Glass, E. M., Goesmann, A., Hanson, A., Iwata-Reuyl, D., Jensen, R., Jamshidi, N., Krause, L., Kubal, M., Larsen, N., Linke, B., McHardy, A. C., Meyer, F., Neuweger, H., Olsen, G., Olson, R., Osterman, A., Portnoy, V., Pusch, G. D., Rodionov, D. A., Ruckert, C., Steiner, J., Stevens, R., Thiele, I., Vassieva, O., Ye, Y., Zagnitko, O. & Vonstein, V. The subsystems approach to genome annotation and its use in the project to annotate 1000 genomes. Nucleic Acids Res (2005) 33, 5691, doi:10.1093/nar/gki866.
- Thieme, F., Koebnik, R., Bekel, T., Berger, C., Boch, J., Buttner, D., Caldana, C., Gaigalat, L., Goesmann, A., Kay, S., Kirchner, O., Lanz, C., Linke, B., McHardy, A. C., Meyer, F., Mittenhuber, G., Nies, D. H., Niesbach-Klosgen, U., Patschkowski, T., Ruckert, C., Rupp, O., Schneiker, S., Schuster, S. C., Vorholter, F. J., Weber, E., Puhler, A., Bonas, U., Bartels, D. & Kaiser, O. Insights into genome plasticity and pathogenicity of the plant pathogenic bacterium Xanthomonas campestris pv. vesicatoria revealed by the complete genome sequence. J Bacteriol (2005) 187, 7254, doi:10.1128/JB.187.21.7254-7266.2005.
2004
- McHardy, A. C., Goesmann, A., Puhler, A. & Meyer, F. Development of joint application strategies for two microbial gene finders. Bioinformatics (2004) 20, 1622, doi:10.1093/bioinformatics/bth137.
- McHardy, A. C., Puhler, A., Kalinowski, J. & Meyer, F. Comparing expression level-dependent features in codon usage with protein abundance: an analysis of 'predictive proteomics'. Proteomics (2004) 4, 46, doi:10.1002/pmic.200300501.
2003
- Goesmann, A., Linke, B., Rupp, O., Krause, L., Bartels, D., Dondrup, M., McHardy, A. C., Wilke, A., Puhler, A. & Meyer, F. Building a BRIDGE for the integration of heterogeneous data from functional genomics into a platform for systems biology. J Biotechnol (2003) 106, 157, doi:10.1016/j.jbiotec.2003.08.007.
- Kalinowski, J., Bathe, B., Bartels, D., Bischoff, N., Bott, M., Burkovski, A., Dusch, N., Eggeling, L., Eikmanns, B. J., Gaigalat, L., Goesmann, A., Hartmann, M., Huthmacher, K., Kramer, R., Linke, B., McHardy, A. C., Meyer, F., Mockel, B., Pfefferle, W., Puhler, A., Rey, D. A., Ruckert, C., Rupp, O., Sahm, H., Wendisch, V. F., Wiegrabe, I. & Tauch, A. The complete Corynebacterium glutamicum ATCC 13032 genome sequence and its impact on the production of L-aspartate-derived amino acids and vitamins. J Biotechnol (2003) 104, 5, doi:10.1016/S0168-1656(03)00154-8.
- McHardy, A. C., Tauch, A., Ruckert, C., Puhler, A. & Kalinowski, J. Genome-based analysis of biosynthetic aminotransferase genes of Corynebacterium glutamicum. J Biotechnol (2003) 104, 229, doi:10.1016/S0168-1656(03)00161-5.
- Meyer, F., Goesmann, A., McHardy, A. C., Bartels, D., Bekel, T., Clausen, J., Kalinowski, J., Linke, B., Rupp, O., Giegerich, R. & Puhler, A. GenDB--an open source genome annotation system for prokaryote genomes. Nucleic Acids Res (2003) 31, 2187, doi: 10.1093/nar/gkg312.
- Wilke, A., Ruckert, C., Bartels, D., Dondrup, M., Goesmann, A., Huser, A. T., Kespohl, S., Linke, B., Mahne, M., McHardy, A. C., Puhler, A. & Meyer, F. Bioinformatics support for high-throughput proteomics. J Biotechnol (2003) 106, 147, doi: 10.1016/j.jbiotec.2003.08.009.
Computational biology of viral pathogens
The Research Department “Computational Biology for Infection Research” at the HZI studies rapidly evolving viral pathogens, such as influenza, hepatitis and human cytomegaloviruses and their coevolution with the adaptive immune response of the human host using computational techniques. A particular focus are influenza A viruses, where we combine epidemiological, genetic, antigenic and structural information on circulating viral strains to determine the antigenicity-altering areas on the protein structure, key sites and amino acid changes and analyze how these affect the viral fitness with regards to escaping human immune response [1,3,5]. In collaboration with infection biologists and immunologists from the HZI, the Hannover Medical School and the German Centre for Infection Research (DZIF), we also study the adaptation of influenza viruses to novel hosts and for maintaining fitness under immune selection in animal models. Viral pathogens such as hepatitis and human cytomegaloviruses differ from influenza viruses in that they cause chronic, not short-term infections. Together with collaborators we track the evolution of these pathogens and the corresponding adaptive immune ‘evolution’ of the host within individual patients over time. We thus aim to generate novel insights into pathogen-host co-evolution and identify leads of translational relevance, such as for development of a universal vaccine against hepatitis C virus infections.
We focus on:
- Computational prediction of suitable vaccine strains for human influenza A
- Determining epitopes for broadly neutralizing antibodies for development of a “universal vaccine” against hepatitis C virus infections
- Reconstructing viral haplotypes from deep sequencing data
- Inference of viral spread trajectories with viral phylogeographies
- Development of a universal vaccine against influenza A viruses
SARS-COV-2 resources
VirusTracker
High resolution global spread reconstruction of COVID-19 via air travel from genome and geographic location data available up to mid February 2020 using the method in (Reimering et. al, PLOS Computational Biology, 2020). Interactive visualisations using
https://nextstrain.github.io/auspice/ are available at https://corona.bifo.helmholtz-hzi.de/ncov2.
Collaborators
- Wulf Blankenfeldt, Department of Structure and Functions of Proteins, Helmholtz Centre for Infection Research (HZI), Braunschweig, Germany
- Mohammad Mofrad, Departments of Bioengineering and Mechanical Engineering, University of California, Berkeley, USA
- Gülsah Gabriel, Heinrich Pette Institute, Leibniz Institute for Experimental Virology, Hamburg, Germany
- Carlos Guzmán, Department of Vaccinology and Applied Microbiology, Helmholtz Centre for Infection Research (HZI), Braunschweig, Germany
- Thomas Pietschmann, Institute for Experimental Virology, Twincore Centre for Experimental and Clinical Infection Research, Hannover, Germany (DZIF collaboration)
- Thomas Schulz, Institute of Virology, Hannover Medical School (MHH), Hannover, Germany
- Klaus Schughart, Infection Genetics, Helmholtz Centre for Infection Research (HZI), Braunschweig, Germany
- Thomas Krey, Institute of Virology, Hannover Medical School (MHH), Hannover, Germany
Computational microbiome research
A research focus of BIFO is the study of microbial communities, including bacteria, viruses and eukaryotic community members, and their relevance for human health and disease. The human microbiota is implicated in a variety of diseases and subject of experimental studies at HZI. Direct metagenome, -transcriptome or -proteome sequencing of microbial community samples enables the study of the majority of microorganisms that cannot be obtained in pure culture, corresponding to the vast majority of the microbial world.
Research in BIFO focuses on establishing data-driven computational approaches that further advance individualized infection medicine in the clinic, such as computational biomarker discovery from microbial omics data, i.e. genotype-phenotype and genotype-environment inference, and the data-driven discovery molecular predictors of host disease status and pathogen phenotypes. We also develop methods for common meta’ome data types, and promote the development of standards and best practices via the Initiative for the Critical Assessment of Metagenome Interpretation (CAMI).
BIFO currently focuses on the following problems and questions:
- Can we identify biomarkers for clinically relevant phenotypes from microbiome data using machine learning approaches and reliably predict these phenotypes? This is particularly relevant for the analysis of cost-efficient 16S data, which however does not encode any information about the functional gene repertoire of a sample.
- Which software is particularly well suited for processing different kinds of metagenome samples? A. McHardy founded and organizes (together with A. Sczyrba) CAMI, the Initiative for the Critical Assessment of Metagenome Interpretation, which aims to establish standards and best practices in metagenome analysis by organizing benchmarking challenges for method developers.
- Can we reconstruct the genomes of individual strains from metagenomics data? This question has large clinical relevance, as individual strains of the same species can have very different phenotypes (e.g. the probiotic E. coli Nissle versus the EHEC strain).
- Which traces does the adaptation of microbial communities to a certain environment leave in the microbiome? Specifically we are interested in this question for the human microbiota and for the spread of antibiotic resistances.
- What can we learn about the role of the microbial CRISPR-CAS system in the human microbiome by systematic metagenome analyses combined with deep learning techniques?
Selected publications
Researchers
- Dr. Fernando Meyer
- Dr. Ehsaneddin Asgari
- Dr. Till Robin Lesker
- Dr. Zhiluo Deng
- Adrian Fritz
- Philipp Münch
- Tzu-Hao Kuo
Collaborators
Current:
- Justin O’Grady & Gemma Kay, Quadram Institute, Norwich, UK
- Markus Cornberg, Hannover Medical School, Hannover, Germany
- Thomas Schulz, Hannover Medical School, Hannover, Germany
- Curtis Huttenhower, Harvard T.H. Chan School of Public Health, Boston, MA, U.S.
- Barbara Stecher, Medical Microbiology and Hospital Epidemiology, Max von Pettenkofer Institute, Ludwig Maximilian University of Munich, Munich, Germany
- Phil Pope and Vincent Eijsink, Norwegian University of Life Sciences, Aas, Norway
- Nadine Ziemert, Natural Product Genome Mining, Eberhard Karls University of Tübingen, Tübingen, Germany (DZIF collaboration)
- Alexander Sczyrba, Aaron Darling, Tanja Woyke…and the further CAMI initiative
- Till Strowig, Microbial Immune Regulation, Helmholtz Centre for Infection Research (HZI), Braunschweig, Germany
Past:
- Paul Schulze-Lefert, Max Planck Institute for Plant Breeding Research, Cologne, Germany
- Phil Pope and Vincent Eijsink, Norwegian University of Life Sciences, Aas, Norway
- Johannes Gescher, Institute of Applied Biosciences (IAB), Karlsruhe Institute of Technology (KIT), Karlsruhe, Germany
- Mark Morrison, CSIRO Livestock Industries, Queensland, Australia
- Jeffrey Gordon and Peter Turnbaugh, Center for Genome Sciences, Washington University, St. Louis, Missouri, USA
- Phil Hugenholtz, Australian Center for Ecogenomics, Queensland, Australia
- Isidore Rigoutsos, Computational Medicine Center, Thomas Jefferson University, Philadelphia, Pennsylvania, USA
- Andreas Brune, Research Group Leader, Department of Biogeochemistry, Max Planck Institute for Terrestrial Microbiology, Marburg, Germany
- Mila Chistoserdova, Department of Chemical Engineering, University of Washington, Seattle, Washington, USA
Machine Learning
High-throughput sequencing combined with metagenomics has uncovered thousands of novel bacterial species directly from samples without isolation or culturing. This enables fine-grained analyses of the functions of microbial community members, and the study of their association with phenotypes and environments, as well as studies of microevolution and adaptation to changing environmental conditions. These sequencing efforts led to a sheer amount of data that can be analyzed using machine learning methods and can help to uncover relationships between human health and diseases.
In this research focus we are:
- Developing novel machine learning methods implemented as software libraries that can be used by the research communities.
- Developing efficient machine learning models that utilize the vast amount of unlabeled biological data.
- Applying machine learning methods on microbial datasets to analyze the interaction between bacteria and the mammalian host (see Computational microbiome research).
- Developing machine and deep learning models to predict properties of molecules and identify their most important structural characteristics.
- Developing machine learning methods to predict B-cell epitopes for epitope mapping and designing novel vaccines.
We focus on:
- GenomeNet - A deep neural network for genomic modelling, semi-supervised classification and imputation: The GenomeNet project is a BMBF funded joint research enterprise of the Helmholtz Centre for Infection Research and the University of Munich with close collaboration with the Harvard T.H. Chan School of Public Health. In this project we aim to develop customized deep learning network architectures which are particularly suited for modeling of large nucleotide sequences. These networks will then be employed on bacterial, viral and human genomes with the goal to understand the complex structures underlying the code of life. This work is funded by the Federal Ministry of Education and Research (031L0199A).
- Learning structures in the CRISPR-Cas system using deep learning architectures: In this project we apply statistical models to specify structural properties of CRISPR cassettes. These properties will be used to further describe potential functions and their classification. This work is funded by the Deutsche Forschungsgemeinschaft (405892038).
Selected publications
Collaborators
- Prof. Bernd Bischl, Ludwig Maximilian University of Munich
- Prof. Bärbel Stecher, Ludwig Maximilian University of Munich
- Prof. Curtis Huttenhower, Harvard School of Public Health
- Dr. Eric Franzosa, Harvard School of Public Health
- Volkswagen Lab
Ongoing Grants
2021 “NFDI4Microbiota - National Research Data Infrastructure for Microbiota Research” (DFG)
2021 “GHGA-Microbiota: Increasing the multimodal use of human and microbiome-related omics data” - GHGA Flex Funds Projekt (DFG)
2021 “Hepatitis C Control: Towards prophylaxis and identification of those in need of treatment” (DZIF) (BMBF)
2019 “Paving the way towards individualized vaccination (i.Vacc) - Exploring multi-omics Big Data in the general population based on a digital mHealth cohort” – Volkswagen Stiftung
2019 “Drug discovery and cheminformatics for new anti-infectives (iCA)” – Lower Saxony Doctoral Program
2019 “Rational design of a universal flu vaccine using recombinant neuraminidase” – Global Grand Challenges of the Bill & Melinda Gates Foundation
2019 “GenomeNet: A deep neural network for genomic modelling, semi-supervised classification and imputation” – Computational Life Sciences Call, Bundesministerium für Bildung und Forschung (BMBF)
2019 “RESIST - Resolving Infection Susceptibility“ – Exzellenzcluster 2155, Deutsche Forschungsgemeinschaft (DFG)
2018 “Learning structures in the CRISPR-Cas system using deep learning architectures” – SPP2141 Deutsche Forschungsgemeinschaft (DFG)
2017 “HiGHmed (Heidelberg-Göttingen-Hannover Medizininformatik)“ - Bundesministerium für Bildung und Forschung (BMBF)
2014 “TI Bioressourcen, Biodaten und digitale Gesundheit” (vorher: “TI Bioinformatics Platform“ - Deutsches Zentrum für Infektionsforschung (DZIF) (BMBF)
Concluded Grants
2020 Corona VAC “Proof of concept study of a SARS-COV-2 vaccine based on recombinant spike protein“ - Niedersächsischen Ministerium für Wissenschaft und Kultur (MWK)
2017 “Sparse2Big: Data fusion and imputation from massive sparse data consortium” - Information and Data Science Initiative, Helmholtz Society
2017 “Bioinformatics support for the development of a prophylactic HCV vaccine candidate” - Deutsches Zentrum für Infektionsforschung (DZIF)
2017 “Communities Allied in Infection coalition" - Volkswagen Foundation
2016 “A Method for Tracking CRISPR/Plasmidome Dynamics in Complex Bacterial Communities“ – Research Grant, Deutsche Forschungsgemeinschaft (DFG)
2014 “Isolation and characterization of novel azidophilic archaea (with J. Gescher)” – Deutsche Forschungsgemeinschaft (DFG)
Further Groups of the Department Computational Biology of Infection Research
Host (epi)genomics - Dr Andreas Klötgen
High-throughput technologies such as microarray chips and next-generation sequencing have enabled screening of a wide range of patients in genome-wide association studies, revealing unprecedented insights into genomic, transcriptomic and plenty of different epigenomic drivers of disease.
Transcriptional regulation is a multistep process involving not only the transcription machinery and transcription factors, but is also defined by histone modifications, nuclear chromatin organization and availability of cis-regulatory elements (for example enhancers). We study the composition of such factors with respect to transcriptional output in immune development and infectious diseases 1. To this end, the incorporation of Hi-C data (3D chromatin information) with ChIP-seq of histone modifications and chromatin regulators has enabled us to predict the chromatin environment of leukemia and how targeted drug therapies affect this complex network 2. Furthermore, more recent techniques such as HiChIP require advanced computational approaches not yet fully developed, and have been used to identify the involvement of Klf4 in cellular reprogramming 3.
However, the complexity of protein expression and regulation does not end at gene transcription. Posttranscriptional regulation ranges from controlling mRNA stability to translational rate and more, and can be studied by specific sequencing approaches 4. We study the effects of RNA-binding proteins (RBPs) by projecting miRNA expression and binding onto mRNA expression regulation 5 with the help of PAR-CLIP. Or, study the direct effects of RPBs on mRNA binding and stability, and how such regulators affect downstream cellular processes in disease 6. As in any computational biology field, the development of new approaches for gaining deeper insights into the underlying biology is key 7, and we continue to develop computational methods and integrative pipelines for various purposes and sequencing approaches.
Our research focuses on
- How do chromatin regulators, histone modifications and chromatin organization orchestrate the complex transcription machinery in immune cells? Do these features hold predispositions of their own for infectious diseases?
- Integration of RNA-binding protein information with RNA-seq or miRNA-seq to achieve a more directed way of posttranscriptional gene regulation by individual RBPs
- How can novel machine learning or deep learning approaches support integrative tasks of genomics and transcriptomics data? To this end, we develop computational approaches to deal with novel and custom sequencing data, and to integrate complex data from various sources in order to achieve a complete view of (post-)transcriptional gene regulation.
Selected publications
- A. Kloetgen, P. Thandapani et al., 3D Chromosomal Landscapes in Hematopoiesis and Immunity. Trends Immunol. 2019 40: 809
- A. Kloetgen, P. Thandapani, P. Ntziachristos et al., Three-dimensional chromatin landscapes in T cell acute lymphoblastic leukemia. Nat Genet. 2020 52: 388
- D. C. Di Giammartino, A. Kloetgen, A. Polyzos A et al., KLF4 is involved in the organization and regulation of pluripotency-associated three-dimensional enhancer networks. Nat Cell Biol. 2019 21: 1179
- A. Kloetgen et al., Biochemical and bioinformatic methods for elucidating the role of RNA-protein interactions in posttranscriptional regulation. Brief Funct Genomics. 2015 14 :102
- K. Hezaveh, A. Kloetgen, S. H. Bernhart et al., Alterations of microRNA and microRNA-regulated messenger RNA expression in germinal center B-cell lymphomas determined by integrative sequencing analysis. Haematologica. 2016 101: 1380
- S. Duggimpudi, A. Kloetgen, S. K. Maney et al., Transcriptome-wide analysis uncovers the targets of the RNA-binding protein MSI2 and effects of MSI2's RNA-binding activity on IL-6 signaling. J Biol Chem. 2018 293: 15359
- A. Kloetgen et al., The PARA-suite: PAR-CLIP specific sequence read simulation and processing. PeerJ. 2016 4:e2619
Researchers
- Dr. Andreas Kloetgen
Collaborators
- Abel Viejo-Borbolla, MHH, Hannover, Deutschland
- Effie Apostolou, Weill Cornell Medicine, New York, USA
- Ari Melnick, Weill Cornell Medicine, New York, USA
- Tracy McGaha, Princess Margaret Cancer Centre, Toronto, Kanada
- Iannis Aifantis, NYU Langone Health, New York, USA
Job Offers
The Research Department “Computational Biology for Infection Research” welcomes applications at all seniority levels in the following fields:
- Bioinformatics / Computational Biology
- Computer Science
- Statistics
- Biomathematics
We are looking for motivated applicants with a strong background in the above-mentioned fields, good coding skills and interest in interdisciplinary research in biology and infection research.
Methods that we employ or develop in our research are related to the fields of:
- Bioinformatics
- Machine learning and deep learning
- Phylogenetics and population genetics
The goal of our projects is the development of algorithms and computer-aided methods to analyze the human microbiome, viral and bacterial pathogens, and human cell lineages within individual patients.
Some, but not all, currently open positions are listed below. Therefore unsolicited applications are always welcome. Please send your applications to Jobs.
Open job offers:
Project Manager / Scientific Writer (f/m/d)
Deep Learning for Molecular Biology
The seminar "Deep Learning for molecular biology" is hosted by the department "Computational Biology of Infection Research" at the HZI headed by Prof. Alice McHardy.
Kick-off Meeting: 04 April 2024, 10 a.m.
Room Kick-off Meeting: BRICS, room 207
Seminar Date: TBA
Room seminar: TBA
Max. number of participants: 10
Language: English
Modus: 30 minutes of presentation (including discussion) + 3-5 pages written summary
Designated for Bachelor and Master Students of Computer and Data Science
Prerequisites: Familiarity with programming in Python and Linear Algebra (matrix / vector multiplications)
In case you have questions about the seminar, contact Mohammad Hadi Foroughmand Araabi.
Description: Recently, deep neural network models have revolutionized machine learning research and achieved state-of-the-art performance in almost every related research, including computer vision, natural language processing, and computational biology. The goal of this seminar is to teach the basic principles of deep learning along with some basic implementations in pytorch framework. We will explore the most fundamental neural architectures including convolutional neural networks, recurrent neural networks, and autoencoders as well as language-model based representation learning methods.
Topics:
- Deep Neural Networks and back propagation
- Convolutional Neural Networks (CNN)
- Recurrent Neural Networks (RNN)
- Representation Learning